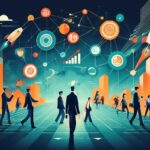
AI in B2B Marketing: Targeting the Right Accounts Effectively
March 18, 2025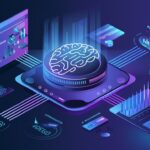
How AI Enables Real-Time Personalization for Websites
March 20, 2025In today’s fast-paced digital landscape, businesses face an overwhelming number of leads daily. However, not all leads hold the same potential for conversion, making it essential to identify the most promising ones efficiently. Traditional lead scoring methods, which rely on manual assessment and basic rule-based models, often fall short in accuracy and scalability. This is where Artificial Intelligence (AI) revolutionizes lead management.
AI-powered lead scoring and prioritization leverage machine learning, predictive analytics, and data-driven insights to rank leads based on their likelihood to convert. By automating and enhancing the lead evaluation process, AI ensures that sales teams focus their efforts on high-value prospects, leading to improved conversion rates, reduced sales cycles, and optimized resource allocation. This article explores how AI is transforming lead scoring and prioritization, covering its benefits, challenges, key technologies, and best practices for implementation.
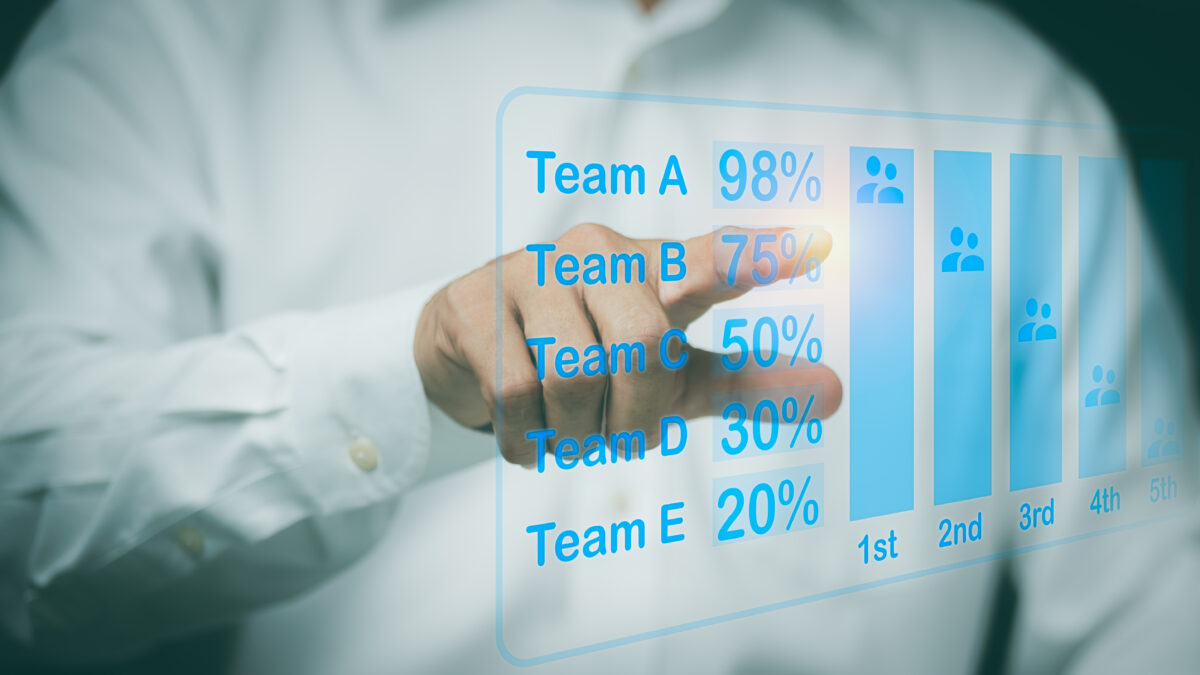
2. Understanding Lead Scoring
Lead scoring is the process of assigning a numerical value to potential customers based on their likelihood of converting into paying clients. Traditionally, businesses relied on manual or rule-based lead scoring, where sales and marketing teams assigned points based on predefined criteria such as demographics, firmographics, website visits, and email engagement. However, this approach has significant limitations.
Manual lead scoring is prone to biases and inconsistencies, as it depends on subjective human judgment. It also lacks scalability, making it inefficient for handling large volumes of leads. Furthermore, static scoring models fail to adapt to changing customer behavior and evolving market trends. In contrast, AI-driven lead scoring overcomes these challenges by dynamically analyzing vast datasets and identifying patterns that indicate high purchase intent. AI learns from past interactions and continuously refines its scoring criteria, resulting in more precise and actionable insights.
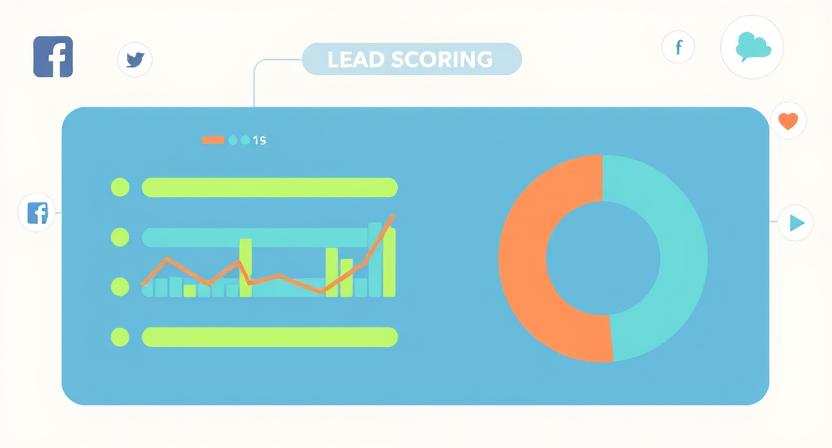
3. The Role of AI in Lead Scoring
AI revolutionizes lead scoring by automating data collection, analysis, and ranking through advanced algorithms. Unlike traditional methods, AI-powered lead scoring relies on machine learning models that evaluate multiple data points simultaneously to determine lead quality. AI-driven models assess both explicit and implicit lead attributes, such as job titles, industry, browsing behavior, social media activity, and email response rates.
Predictive analytics plays a crucial role in AI-powered lead scoring. By examining historical sales data, AI can predict which leads are most likely to convert. These models analyze past successful conversions to identify common characteristics among high-quality leads, allowing businesses to refine their outreach strategies. Additionally, AI can process unstructured data, such as text from emails or social media conversations, using natural language processing (NLP) to extract meaningful insights.
Another advantage of AI is real-time lead qualification. Traditional lead scoring models often operate on static data, meaning they may fail to detect sudden changes in a prospect’s behavior. AI continuously updates lead scores based on the latest interactions, ensuring that sales teams always have the most accurate and up-to-date information when prioritizing outreach efforts.
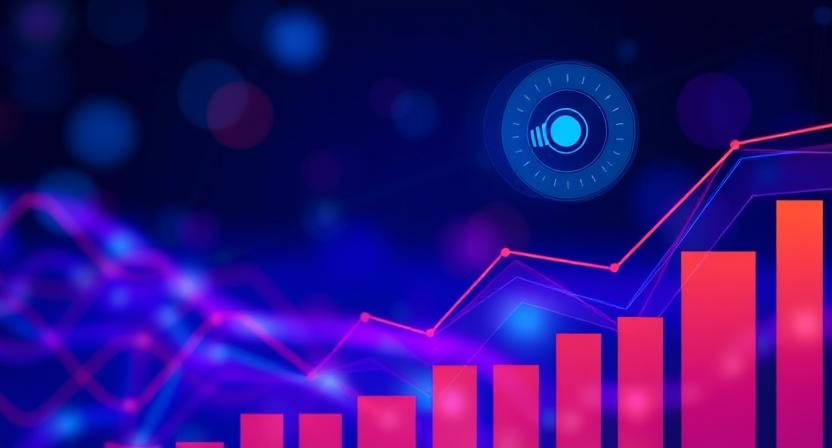
4. How AI Enhances Lead Prioritization
Beyond scoring, AI also improves lead prioritization by ranking leads based on their conversion potential and urgency. By analyzing engagement levels, AI can differentiate between passive leads and those showing strong buying signals. For instance, if a lead frequently visits a company’s pricing page, downloads whitepapers, or engages with email campaigns, AI will assign them a higher priority.
AI also enhances personalization, which is a key factor in effective lead prioritization. By understanding individual customer preferences and pain points, AI enables businesses to tailor their messaging and outreach strategies. This level of customization significantly increases the chances of converting high-priority leads.
Furthermore, AI automates follow-up strategies by determining the optimal time and channel for outreach. For example, AI can analyze past communication patterns and suggest whether an email, phone call, or LinkedIn message would be most effective. It can also automate follow-ups by sending personalized messages based on lead behavior, ensuring no high-quality lead is neglected due to human oversight.
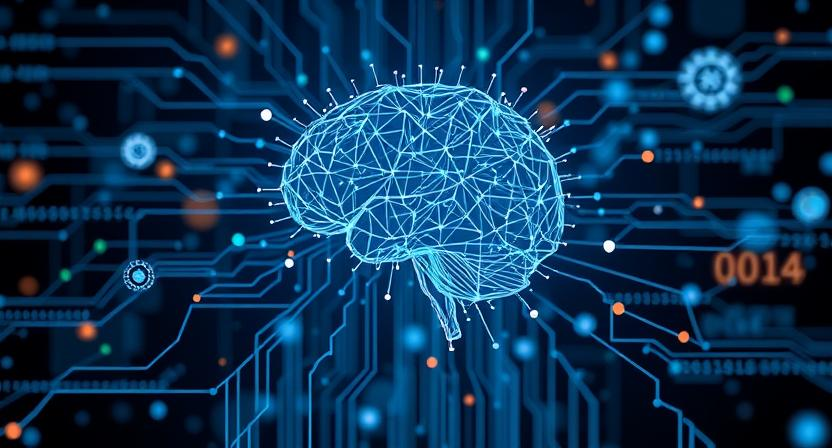
5. Key AI Technologies for Lead Scoring
Several AI technologies contribute to the effectiveness of lead scoring and prioritization. Machine learning (ML) algorithms form the foundation of AI-powered lead scoring by identifying patterns and predicting conversion probabilities based on historical data. Deep learning models take this a step further by analyzing complex, non-linear relationships between different lead attributes.
Natural language processing (NLP) enables AI to analyze textual data from various sources, including customer emails, chatbot interactions, and social media conversations. By extracting sentiments, intent, and key phrases, NLP helps determine a lead’s level of interest and readiness to buy.
Predictive analytics uses statistical models and machine learning techniques to forecast future outcomes based on historical data. In the context of lead scoring, predictive analytics identifies the most promising leads by evaluating their past interactions, behaviors, and engagement levels.
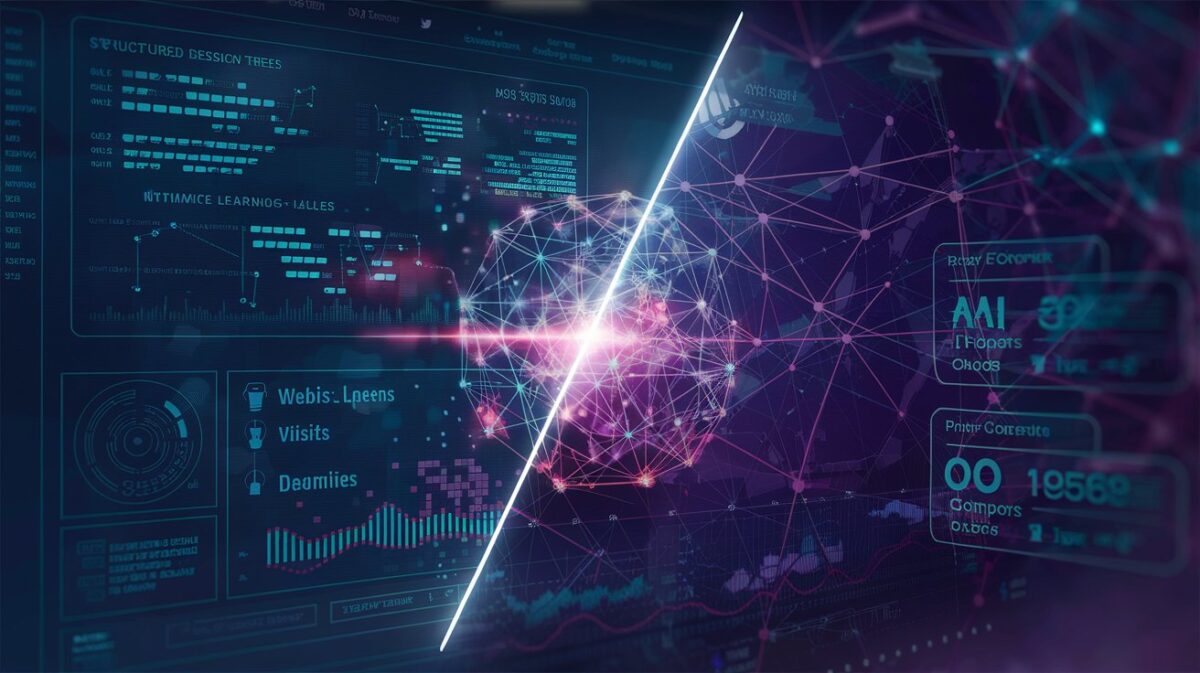
6. AI-Based Lead Scoring Models
AI-powered lead scoring can be implemented using different models, each with its unique approach. Explicit scoring models assign points based on predefined criteria, such as job role, company size, and geographic location. Implicit scoring models, on the other hand, focus on behavioral indicators, such as website visits, content downloads, and email open rates.
Rule-based scoring models follow a set of predetermined conditions, such as “if a lead opens an email three times, assign 10 points.” While useful for basic automation, these models lack adaptability. Predictive scoring models, driven by machine learning, analyze vast datasets to determine which factors are most indicative of conversion. These models continuously learn and refine their criteria based on real-world outcomes.
Common AI techniques used in lead scoring include logistic regression, decision trees, random forests, and neural networks. Each technique varies in complexity and interpretability, with more advanced models offering higher accuracy at the cost of transparency.
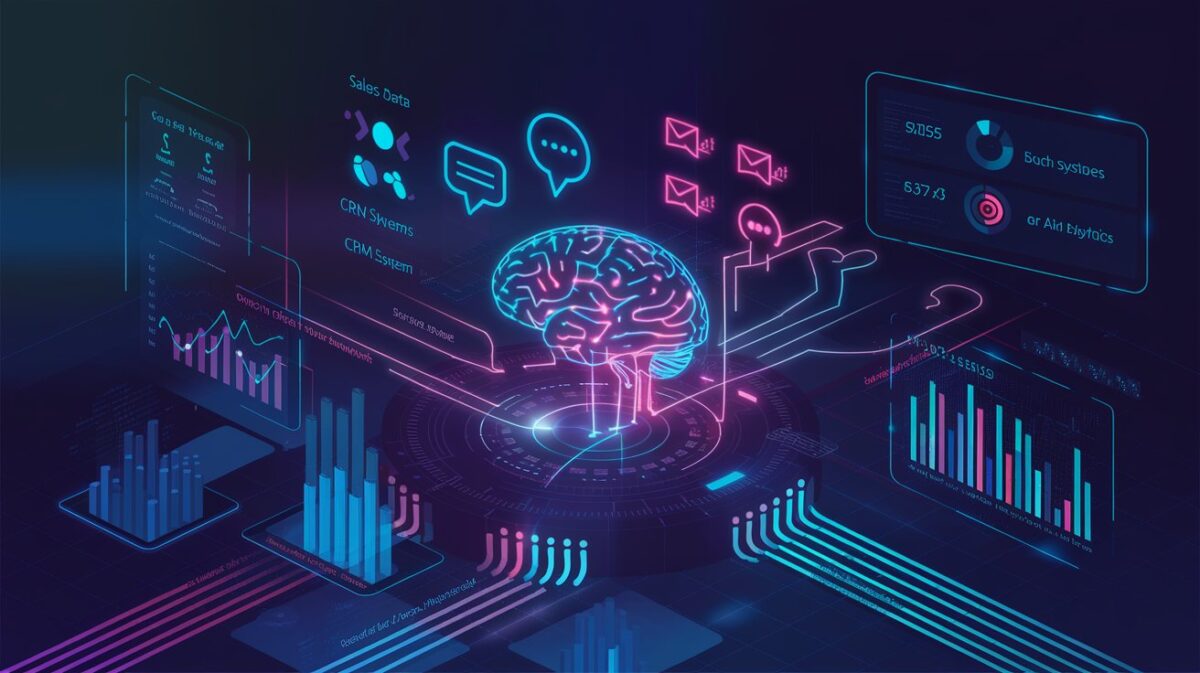
7. Data Sources for AI-Powered Lead Scoring
AI-driven lead scoring requires access to multiple data sources to generate accurate insights. Customer relationship management (CRM) data is a primary input, as it contains valuable information on past interactions, sales history, and customer preferences. AI integrates with CRM platforms to analyze customer data and identify high-value leads.
Other essential data sources include web analytics, social media activity, and email engagement. AI can track website behavior, such as time spent on product pages and frequency of visits, to assess a lead’s interest level. Social media interactions, such as likes, comments, and shares, provide additional indicators of engagement. Email engagement metrics, including open rates, click-through rates, and response rates, further refine lead scoring accuracy.
Additionally, AI can leverage third-party data enrichment tools to gather supplementary information on leads, such as company financials, industry trends, and competitor analysis. These insights help sales teams gain a deeper understanding of their prospects and tailor their approach accordingly.
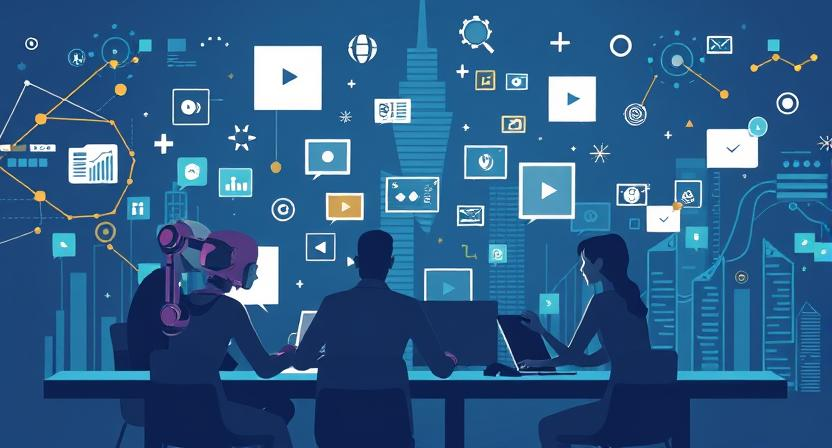
8. Benefits of AI in Lead Scoring and Prioritization
AI-powered lead scoring and prioritization bring a multitude of advantages that significantly enhance the efficiency and effectiveness of sales and marketing teams. One of the most notable benefits is increased accuracy. Traditional lead scoring methods rely on static rules that may not reflect real-time customer behavior. In contrast, AI continuously refines its scoring models based on real-time data, leading to more precise lead evaluations.
Another major advantage is the ability to process vast amounts of data from multiple sources. AI integrates information from CRM systems, website interactions, email engagement, and social media activity to build a comprehensive profile of each lead. This holistic approach ensures that businesses do not overlook important signals that indicate a lead’s readiness to convert.
AI-driven lead scoring also results in better resource allocation. Sales teams can prioritize high-value leads while reducing time spent on low-quality prospects. This leads to shorter sales cycles and higher conversion rates, ultimately increasing revenue. Furthermore, AI’s ability to automate lead scoring and prioritization reduces the manual workload for sales teams, allowing them to focus on relationship-building and closing deals rather than sifting through large lists of unqualified leads.
Another significant benefit is improved alignment between sales and marketing teams. AI provides objective, data-driven insights that help both teams work towards common goals. Marketing teams can refine their lead generation strategies based on AI-driven feedback, while sales teams can rely on AI-scored leads to ensure they are engaging with the right prospects at the right time.
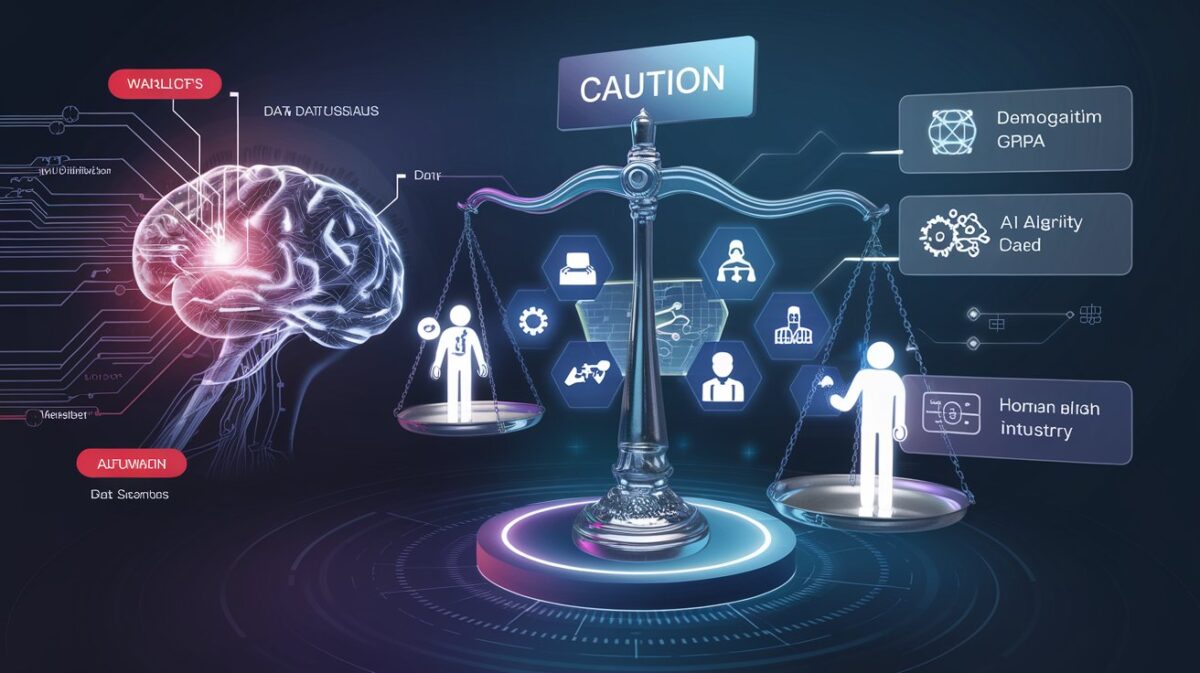
9. Challenges in AI-Powered Lead Scoring
Despite its advantages, AI-powered lead scoring and prioritization come with certain challenges that businesses must address to maximize effectiveness. One of the biggest challenges is data quality. AI models rely on high-quality, accurate, and up-to-date data to make reliable predictions. If the input data contains errors, inconsistencies, or biases, the AI-generated lead scores may be inaccurate. Therefore, businesses must invest in data cleansing and management strategies to ensure the reliability of AI-driven insights.
Another concern is the potential for bias in AI models. AI learns from historical data, and if past lead conversion patterns reflect biases—such as favoring certain industries or demographics—the AI may unintentionally reinforce these biases. To mitigate this risk, businesses should regularly audit their AI models and adjust them to promote fairness and inclusivity.
Balancing automation with human intuition is another challenge. While AI can process vast amounts of data more efficiently than humans, it lacks the human touch required to understand nuanced situations. Some leads may not fit the AI’s predefined criteria but could still be valuable prospects based on qualitative factors. For this reason, businesses should use AI as a supportive tool rather than a complete replacement for human decision-making.
Additionally, companies must ensure compliance with data privacy regulations such as GDPR and CCPA. AI-driven lead scoring often involves analyzing large amounts of customer data, making it essential for businesses to follow ethical data collection and processing practices. Implementing transparent data usage policies and obtaining explicit customer consent can help maintain compliance and build trust with prospects.
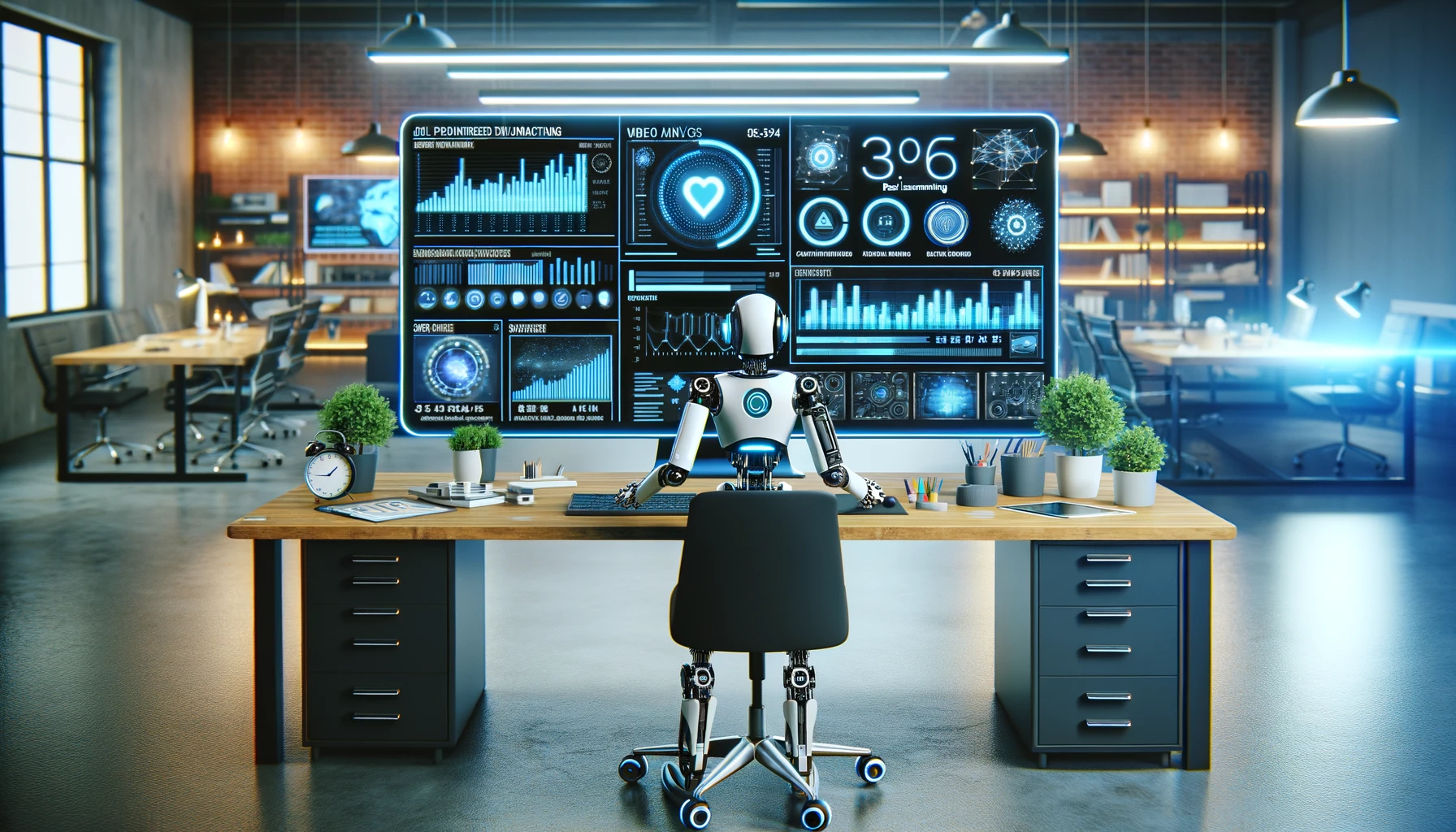
Best Practices for Implementing AI in Lead Scoring
To successfully implement AI-powered lead scoring, businesses should follow a set of best practices to ensure effectiveness and accuracy. The first step is selecting the right AI tools that align with business goals and technical capabilities. Several AI-driven lead scoring platforms are available, including Salesforce Einstein, HubSpot, and Marketo, each offering unique features for different business needs.
Training AI models with relevant and high-quality data is essential for accuracy. Businesses should ensure that their CRM and customer interaction data are clean, consistent, and comprehensive. The more data AI models have to learn from, the better they will become at identifying high-potential leads.
Continuous monitoring and optimization are also crucial. AI models should not be treated as static systems; instead, businesses should regularly review their performance, refine algorithms, and update lead scoring criteria based on evolving market trends and customer behavior. Implementing A/B testing and feedback loops can help fine-tune AI-driven scoring models to improve their predictive accuracy.
Furthermore, integrating AI-powered lead scoring with other marketing and sales tools enhances its effectiveness. AI should work seamlessly with CRM platforms, email marketing software, and sales automation tools to ensure a streamlined workflow. This integration allows businesses to track lead progression in real time and adjust their strategies accordingly.
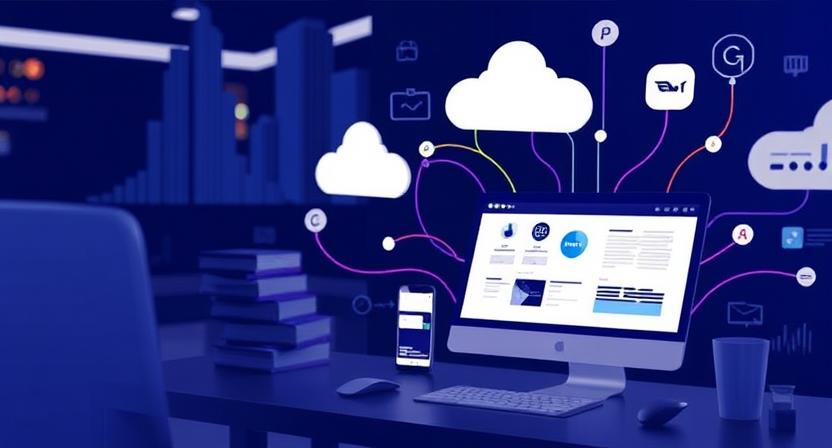
Case Studies: AI-Driven Lead Scoring Success Stories
Several companies across different industries have successfully implemented AI-powered lead scoring to improve their conversion rates and sales efficiency.
For example, a SaaS (Software-as-a-Service) company struggling with high lead volume but low conversion rates implemented AI-driven predictive scoring to prioritize leads. By analyzing past customer data and engagement patterns, the AI model identified key traits that correlated with high-value customers. As a result, the sales team focused their efforts on the most promising leads, leading to a 30% increase in conversions.
In the e-commerce sector, an online retail company used AI-based lead scoring to predict which customers were most likely to make a purchase. AI analyzed factors such as browsing history, abandoned carts, and email interactions to rank potential buyers. By personalizing marketing campaigns based on AI-driven insights, the company saw a significant boost in sales and customer engagement.
Similarly, in the real estate industry, AI-powered lead scoring helped agents identify serious home buyers based on online search behavior, property inquiries, and financial qualifications. This enabled agents to prioritize high-intent leads, reducing the time spent on unqualified prospects and increasing successful transactions.
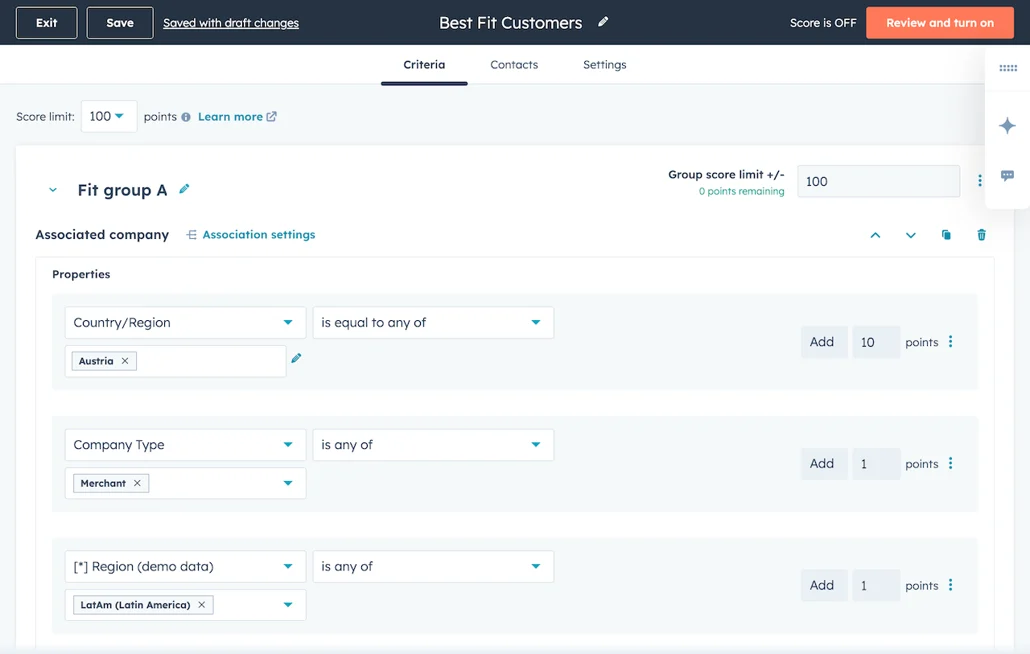
AI Tools for Lead Scoring and Prioritization
Various AI-powered tools and platforms are available to help businesses implement lead scoring effectively. Some of the most popular AI-driven lead scoring solutions include:
- Salesforce Einstein: A powerful AI tool integrated into Salesforce CRM that analyzes past sales data to predict lead conversion probabilities.
- HubSpot Predictive Lead Scoring: Uses machine learning to assess lead quality based on engagement levels and customer behavior.
- Marketo Engage: Offers AI-driven scoring models that help marketing teams identify and prioritize high-value leads.
- InsideSales Predictive Playbooks: Uses AI to optimize sales outreach by ranking leads based on likelihood to convert.
Each of these tools comes with unique features tailored to different business needs. Companies should evaluate pricing, scalability, and ease of integration when selecting the right AI solution for their lead scoring strategy.
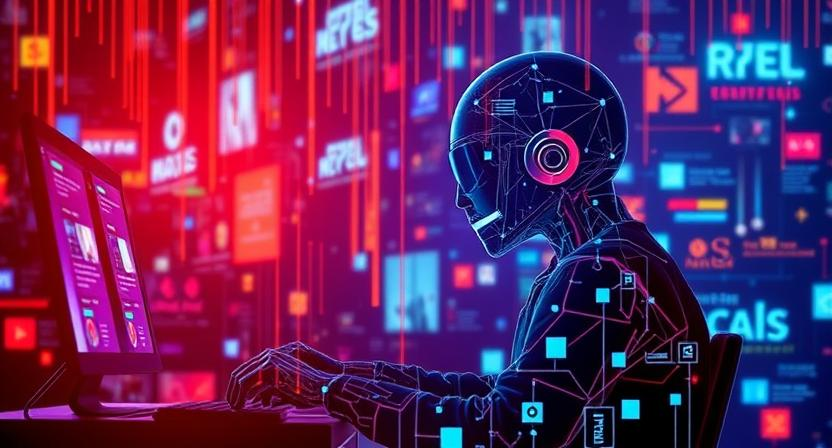
The Future of AI in Lead Scoring
The future of AI in lead scoring is promising, with emerging technologies expected to enhance predictive capabilities further. One major trend is hyper-personalization, where AI not only scores leads but also tailors engagement strategies based on individual customer preferences. By leveraging AI-driven behavioral insights, businesses can create highly customized marketing campaigns that resonate with specific audiences.
Another exciting development is AI-powered intent prediction, which goes beyond traditional lead scoring by identifying prospects who are actively looking to purchase. By analyzing real-time signals such as search queries, competitor interactions, and online content consumption, AI can predict a lead’s intent even before they directly engage with a company.
Additionally, AI will become increasingly integrated with omnichannel marketing strategies, ensuring a seamless customer journey across multiple touchpoints. AI-driven insights will help businesses align their email marketing, social media outreach, and website interactions to provide a consistent and personalized experience.
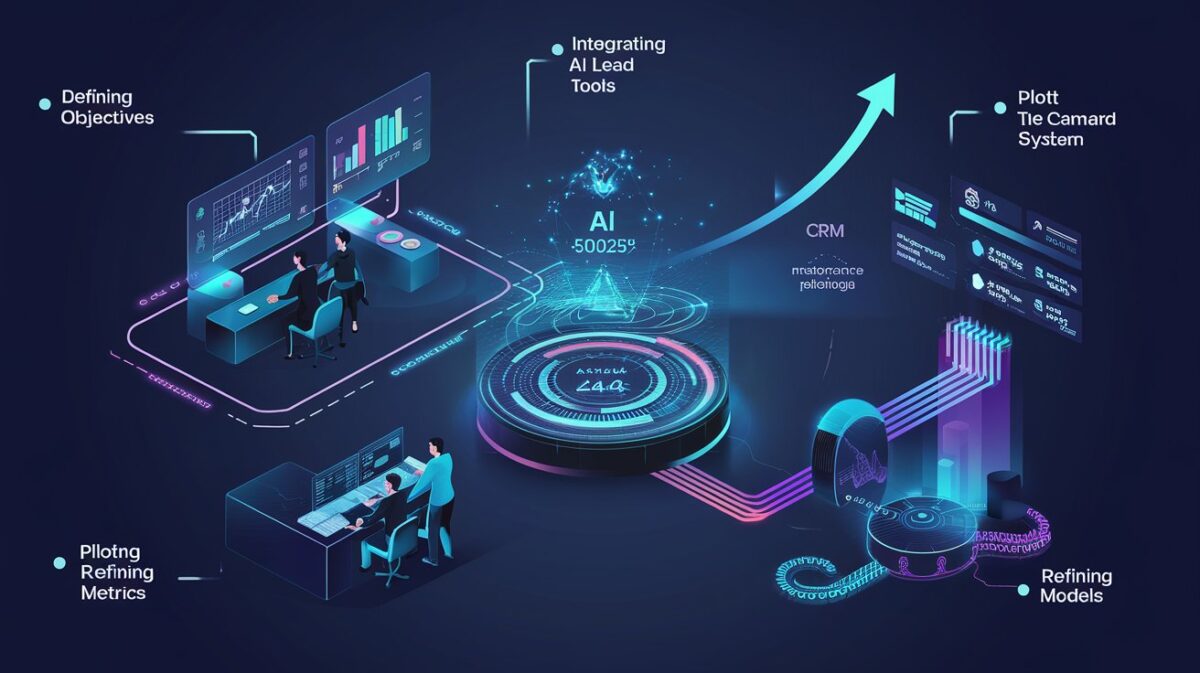
How to Get Started with AI for Lead Scoring
For businesses looking to adopt AI-powered lead scoring, the first step is to define their objectives and key performance indicators (KPIs). Companies should determine what they want to achieve—whether it’s increasing conversion rates, shortening sales cycles, or improving lead qualification accuracy.
Next, businesses should invest in the right AI tools and ensure proper data integration across CRM and marketing platforms. Starting with a small-scale pilot program can help assess AI’s impact before a full-scale rollout.
Finally, businesses should continuously refine their AI models and monitor performance to ensure optimal results. Regularly updating lead scoring criteria based on market changes and customer behavior ensures that AI remains effective in identifying high-value prospects.
Conclusion
AI-powered lead scoring and prioritization have transformed the way businesses identify and engage with potential customers. By leveraging machine learning, predictive analytics, and real-time data analysis, AI enables sales and marketing teams to focus on high-quality leads, improving efficiency and conversion rates. While challenges such as data quality, bias, and compliance must be addressed, businesses that implement AI-driven lead scoring effectively will gain a significant competitive advantage in today’s digital marketplace.