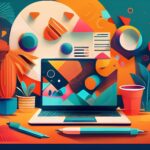
Emotion Analysis in AI Marketing: Decoding Customer Sentiments for Enhanced Campaign Success
September 5, 2023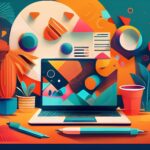
Cultivating Engagement: The Power of Interactive Ads in AI Marketing
September 7, 2023In today’s digital age, where information flows freely, and transactions happen at the speed of light, the battle against fraud has become increasingly complex. Fraudsters are constantly evolving their tactics to deceive individuals and organizations. Fortunately, the advancement of Artificial Intelligence (AI) has opened up new possibilities for detecting and preventing fraud. This comprehensive blog post will delve deep into AI marketing and how it empowers fraud detection. So, fasten your seatbelts as we embark on this journey to unravel the secrets of catching deceptive moves.
Introduction to AI Marketing
Understanding AI in Marketing
Artificial Intelligence (AI) in marketing is not just a trendy buzzword; it’s a technological marvel that has reshaped how businesses engage with their customers. At its core, AI in marketing utilizes machine learning algorithms to process vast amounts of data and extract valuable insights. These insights are then used to tailor marketing strategies and campaigns to specific audiences, enhancing the overall customer experience.
AI-powered marketing tools are like having a team of data scientists working around the clock. They can analyze customer behaviour, preferences, and purchase history to create personalized content and product recommendations. This level of personalization leads to higher customer satisfaction and increased conversion rates.
The beauty of AI lies in its ability to process data at an unprecedented speed. While a human marketer might take hours or even days to analyze customer data, AI algorithms can do it in seconds. This speed allows businesses to respond to real-time market changes, ensuring that their marketing strategies remain relevant and effective.
The Role of AI in Modern Businesses
In the digital age, businesses are swimming in a sea of data. From website analytics to social media interactions, abundant information is waiting to be harnessed. AI is the lifeguard, helping organizations understand and turn this data into actionable insights.
One of the critical roles of AI in modern businesses is automation. Repetitive tasks that used to consume valuable time and resources can now be automated. For example, AI-powered chatbots can handle customer inquiries 24/7, allowing human employees to focus on more complex tasks. This improves efficiency and enhances the customer experience by providing instant responses.
Moreover, AI plays a pivotal role in predictive analytics. By analyzing historical data, AI can forecast future trends and customer behaviour. This foresight allows businesses to make informed decisions, whether it’s adjusting inventory levels, optimizing pricing strategies, or launching targeted marketing campaigns.
Why Fraud Detection Matters in the Digital Age
In the digital age, where online transactions have become the norm, the stakes for fraud detection have never been higher. Fraudsters have adapted to the digital landscape, using sophisticated tactics to deceive individuals and organizations.
AI marketing’s relevance to fraud detection lies in its ability to identify anomalies and patterns that may indicate fraudulent activity. Traditional fraud detection methods often rely on static rule sets, which cunning criminals can easily outsmart. In contrast, AI continuously learns and evolves, making it a formidable opponent in the battle against fraud.
The financial consequences of fraud are significant. For businesses, it can result in substantial monetary losses, damage to reputation, and legal repercussions. For individuals, it can lead to identity theft and financial ruin. Therefore, the ability to detect and prevent fraud is not just a luxury but a necessity in the digital age.
The Evolution of Fraud Techniques
Traditional Fraud Methods
Fraud has been a part of human history for centuries. Traditional fraud methods include counterfeiting, embezzlement, and Ponzi schemes. These tactics rely on deceiving individuals through various means, from forged documents to elaborate confidence tricks.
Historically, combating traditional fraud was challenging but relatively straightforward. Law enforcement and financial institutions developed protocols and safeguards to detect and prosecute these types of fraud. However, with the advent of the digital age, fraudsters found new avenues for deception.
Emergence of Digital Fraud
The digital realm has opened up a vast playground for fraudsters. Digital fraud includes phishing, identity theft, online scams, and ransomware attacks. Criminals can now operate from anywhere worldwide, making it difficult for law enforcement to track them down.
Phishing, for example, involves sending deceptive emails or messages that appear to come from legitimate sources, tricking individuals into revealing sensitive information like login credentials or credit card numbers. On the other hand, identity theft can lead to the unauthorized use of personal information for financial gain.
The Cat-and-Mouse Game
As fraudsters’ techniques evolve, so must the methods used to combat them. This ongoing battle creates a cat-and-mouse dynamic, where each side constantly adapts to the other’s tactics.
Fraudsters are quick to exploit new technologies. For instance, the rise of cryptocurrencies has opened up new opportunities for money laundering and ransomware attacks. They constantly seek weaknesses in systems and exploit them before countermeasures can be implemented.
In response, law enforcement agencies, businesses, and cybersecurity experts work tirelessly to stay ahead of fraudsters. They employ advanced technologies such as AI and machine learning to analyze data and detect unusual patterns that may indicate fraudulent activity.
This constant adaptation and innovation on both sides of the battle highlight the need for robust fraud detection systems, and this is where AI marketing steps in as a powerful ally in the fight against digital fraud.
AI and Machine Learning in Fraud Detection
How AI Algorithms Work
The heart of AI’s effectiveness in fraud detection lies in its algorithms. These algorithms analyze vast amounts of data in real time, searching for patterns and anomalies. Unlike traditional rule-based systems, AI algorithms can sift through immense datasets within milliseconds, detecting irregularities that would be virtually impossible for a human to spot.
One of the critical strengths of AI algorithms is their ability to learn and adapt. They can recognize patterns in historical data and use this knowledge to improve their accuracy over time. This self-improvement aspect is a game-changer in the ever-evolving landscape of fraud.
Benefits of AI in Fraud Detection
The benefits of AI in fraud detection are multifaceted and profound. Firstly, AI can detect fraud in real time, minimizing the damage caused by fraudulent activities. This rapid response can prevent criminals from making off with ill-gotten gains.
Secondly, AI systems reduce the burden on human analysts. By automating the initial stages of fraud detection, these professionals can focus on more complex tasks, such as investigating emerging threats and fine-tuning fraud detection models.
Additionally, AI is exceptionally effective at detecting subtle and complex fraud patterns. It can identify trends and anomalies that would go unnoticed by traditional methods. This capability is particularly valuable in identifying sophisticated fraud schemes.
Challenges in Fraud Detection
False Positives and Negatives
False positives and false negatives are two significant challenges in fraud detection. False positives occur when legitimate transactions or activities are incorrectly flagged as fraudulent. This can lead to inconveniences for customers and damage a business’s reputation.
Conversely, false negatives occur when fraudulent activities go undetected. This can result in financial losses and legal liabilities. Striking the right balance between minimizing false positives and false negatives is an ongoing challenge for fraud detection systems.
Data Privacy Concerns
As AI-powered fraud detection systems collect and analyze vast amounts of data, data privacy and security concerns have come to the forefront. Organizations must ensure that user data is protected and used responsibly to avoid regulatory penalties and reputational damage.
Stringent data privacy regulations, such as the European Union’s General Data Protection Regulation (GDPR), require organizations to be transparent about their data collection and processing practices. This places an added responsibility on businesses to implement robust data protection measures.
Adapting to New Fraud Schemes
Fraudsters are not stagnant; they continually devise new and innovative schemes to bypass detection systems. This dynamic nature of fraud requires fraud detection tools to adapt rapidly.
AI’s ability to learn and evolve makes it well-suited for this challenge. AI can stay one step ahead of fraudsters by continuously analyzing new data and identifying emerging patterns. However, this adaptability also demands ongoing monitoring and updates to AI models to ensure they remain effective.
AI-Powered Tools for Fraud Detection
Fraud Detection Software
Fraud detection software is a critical component of AI-powered fraud detection systems. These software solutions utilize AI algorithms to analyze data and identify anomalies. The flexibility of these tools allows organizations to customize them to their specific needs.
For example, a financial institution may use fraud detection software to monitor transactions for unusual patterns. At the same time, an e-commerce platform may employ the same software to detect fraudulent user accounts and purchases.
Machine Learning Models
Machine learning models are the brains behind AI-powered fraud detection. These models use algorithms to classify transactions and activities as legitimate or fraudulent. They become more accurate over time as they learn from historical data.
Neural networks, decision trees, and support vector machines are examples of machine learning models used in fraud detection. These models excel at recognizing complex patterns and adapting to changing fraud techniques.
Behavioral Analytics
Behavioural analytics is a powerful tool in fraud detection, particularly for identifying insider threats and unusual user behaviour. This approach involves tracking user actions and creating profiles of typical behaviour.
For instance, a company may use behavioural analytics to monitor employee activities on its network. If an employee’s behaviour deviates significantly from their established profile, it could indicate potential misconduct or fraud.
The combination of fraud detection software, machine learning models, and behavioural analytics creates a comprehensive defence against fraudulent activities. However, the successful implementation of these tools requires careful planning and integration into an organization’s existing systems.
Implementing AI Marketing for Fraud Detection
Data Collection and Analysis
Organizations must first focus on data collection and analysis to harness the power of AI marketing for fraud detection. This involves gathering data from various sources, such as transaction records, user behaviour logs, and external threat intelligence feeds.
The quality and quantity of data play a crucial role in the effectiveness of AI-powered fraud detection systems. Clean and comprehensive data sets provide a solid foundation for training machine learning models to recognize fraudulent patterns.
Integration with Existing Systems
The seamless integration of AI marketing tools for fraud detection into an organization’s existing systems is vital for real-time monitoring and response. Whether it’s an e-commerce platform, a financial institution, or a healthcare provider, AI must work with other software and infrastructure.
For example, an e-commerce platform might integrate AI fraud detection into its payment gateway, allowing it to analyze transactions as they occur and flag any suspicious activity. This integration ensures that fraudulent transactions are blocked or flagged for further review before they can cause financial harm.
Training and Fine-Tuning
AI models are not static; they require continuous training and fine-tuning to remain effective. Training involves feeding the model with labelled data, allowing it to learn from past fraud cases and legitimate transactions.
Fine-tuning is an ongoing process that ensures the model adapts to new fraud schemes and evolving patterns. As fraudsters develop new tactics, AI models must learn to recognize these emerging threats.
Implementing AI marketing for fraud detection is not a one-time endeavour but an ongoing commitment to enhancing security and protecting against fraud in the digital age.
The Future of AI Marketing in Fraud Detection
AI-Driven Predictive Analysis
The future of AI marketing in fraud detection holds immense promise. Powered by AI, predictive analysis is set to revolutionize how organizations combat fraud. Rather than merely detecting fraud after the fact, predictive analysis aims to anticipate and prevent it before it happens.
AI can analyze historical data, user behaviour, and market trends to identify potential risks and vulnerabilities. By proactively addressing these issues, organizations can stay one step ahead of fraudsters and minimize their impact.
Predictive analysis also enables businesses to allocate resources more effectively. Instead of reacting to every potential threat, they can focus on the most high-risk areas, optimizing their fraud prevention efforts.
Enhanced User Authentication
User authentication is a critical aspect of fraud prevention. AI-driven authentication methods are on the horizon, offering enhanced security while reducing friction for legitimate users.
For instance, biometric authentication, such as facial recognition and fingerprint scanning, is becoming increasingly prevalent. AI algorithms can analyze biometric data to verify a user’s identity with high accuracy. This eliminates the need for cumbersome passwords and PINs, which can be vulnerable to theft or social engineering.
Furthermore, AI can continuously monitor user behaviour to identify anomalies. If a user’s behaviour deviates significantly from their established patterns, the system can trigger additional authentication measures, such as multi-factor authentication.
In conclusion, AI marketing’s role in fraud detection is a transformative force in the digital age. Its ability to process data quickly, adapt to new fraud schemes, and anticipate threats sets it apart as a crucial tool for organizations and law enforcement agencies. As technology continues to evolve, so too will the capabilities of AI marketing in the ongoing battle against fraud. The digital age may present new challenges, but with AI by our side, we are better equipped than ever to catch deceptive moves and protect our digital world.
FAQs
Q1: What is the main advantage of AI in fraud detection? The primary advantage of AI in fraud detection is its ability to analyze vast amounts of data in real time, detecting patterns and anomalies that would be challenging for humans to identify. This speed and accuracy enable AI to remember fraudulent activities as they occur, minimizing financial losses and damage to reputation.
Q2: How does AI-powered fraud detection differ from traditional rule-based systems? AI-powered fraud detection uses machine learning algorithms to adapt and evolve. It can learn from historical data and recognize emerging fraud patterns, whereas traditional rule-based systems rely on static rules that may become obsolete as fraudsters develop new tactics.
Q3: What are some real-life examples of AI in fraud detection? AI is widely used in fraud detection across various industries. For instance, AI can detect credit card fraud in the financial sector by analyzing transaction patterns. In healthcare, it can identify anomalies in insurance claims. E-commerce platforms use AI to spot unusual user behaviour, such as rapid account creation and high-value orders.
Q4: What are the challenges in implementing AI marketing for fraud detection? Implementing AI marketing for fraud detection requires careful data collection and analysis, integration with existing systems, and ongoing training and fine-tuning of AI models. Organizations must also address data privacy concerns and adapt to new fraud schemes as they emerge.
Q5: What is the future of AI marketing in fraud detection? The future of AI marketing in fraud detection holds promise in predictive analysis and enhanced user authentication. Predictive analysis aims to proactively prevent fraud by identifying potential risks and vulnerabilities. Improved user authentication methods, driven by AI, offer increased security and convenience, reducing the reliance on traditional passwords and PINs.