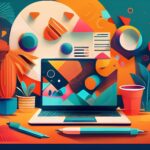
Unveiling the Power of Competitor Analysis in B2B Lead Generation
September 19, 2023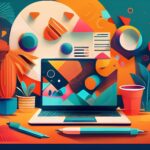
Revolutionizing Content Creation: The Power of AI Marketing
September 20, 2023The Complexity of Retail Inventory
Retail inventory management is a multifaceted endeavor that demands a keen understanding of market dynamics, consumer behavior, and supply chain intricacies. The sheer complexity of retail inventory stems from the fact that it operates within an environment marked by constant change and uncertainty.
Consider a clothing retailer, for instance. They must juggle a diverse range of products, each subject to fluctuating demand influenced by seasons, trends, and even unforeseeable factors like a sudden celebrity endorsement. This makes predicting which items will fly off the shelves and which will linger a daunting task.
Moreover, the inventory manager must grapple with supplier constraints, lead times, and the delicate balance between overstocking and understocking. Overstocking ties up capital, leads to costly storage expenses, and may result in unsold items that eventually need to be discounted. Understocking, on the other hand, results in lost sales and dissatisfied customers.
The Consequences of Poor Inventory Management
The repercussions of poor inventory management can be severe. Overstocking is akin to financial ballast, weighing down a retailer’s profitability. It ties up precious capital that could be invested elsewhere and increases the risk of obsolescence as products languish on the shelves. Additionally, the cost of storing excess inventory in warehouses can be substantial.
Conversely, understocking can lead to stockouts, disgruntled customers, and missed revenue opportunities. When a customer walks into a store or visits an online shop and finds their desired product out of stock, they may not return. This lost business can be challenging to quantify but is undoubtedly detrimental.
How AI Can Revolutionize Inventory Management
In this labyrinth of retail inventory, Artificial Intelligence emerges as a powerful ally. AI algorithms have the capacity to process vast amounts of data, enabling them to make sense of the chaos. They can analyze historical sales data, identify patterns and trends, and, most crucially, predict future demand with remarkable precision.
Imagine an AI system that can crunch data from multiple sources, including past sales, website traffic, social media mentions, weather forecasts, and economic indicators. It synthesizes this information to provide inventory managers with actionable insights. For instance, it can anticipate an uptick in demand for winter coats when the weather forecast predicts a cold front, enabling the retailer to stock up appropriately.
AI doesn’t just forecast demand; it optimizes stock levels. By continually analyzing real-time sales data and adjusting inventory orders accordingly, it helps maintain the delicate balance between excess inventory and stockouts. This is particularly vital in the fast-paced world of fashion, where trends come and go in the blink of an eye.
Real-Life Success Stories
Let’s look at Amazon, the e-commerce juggernaut, as a prime example of AI’s impact on inventory management. Amazon’s recommendation engine, driven by AI, suggests products to customers based on their browsing and purchase history. This not only boosts sales but also refines their demand forecasting algorithms.
Amazon’s warehouses are a marvel of AI integration. Robots whirr around, moving shelves to human pickers, optimizing the retrieval process. AI algorithms control the movement of these robots, ensuring that items are retrieved and shipped with maximum efficiency. This level of automation not only reduces labor costs but also minimizes the time between order placement and delivery, enhancing the customer experience.
In essence, AI transforms inventory management from a guessing game into a data-driven science. It empowers retailers to make informed decisions, reducing costs, minimizing risks, and, most importantly, satisfying customers’ ever-evolving demands.
AI-Driven Demand Forecasting
The Importance of Accurate Demand Forecasting
Demand forecasting is the compass that guides inventory management. An accurate forecast ensures that a retailer has the right products in the right quantities at the right time. It prevents the costly pitfalls of overstocking and understocking.
For a moment, picture a grocery store. Accurate demand forecasting ensures that there are enough turkeys on the shelves for Thanksgiving and sufficient watermelons in the summer heat. If these forecasts are off, it can result in frustrated shoppers and lost sales opportunities.
How AI Enhances Demand Forecasting
AI brings a level of sophistication to demand forecasting that was previously unattainable. Traditional methods relied on historical sales data and basic seasonality patterns. AI, however, goes several steps further.
It considers a multitude of variables, both internal and external. Internal variables might include historical sales, inventory levels, and marketing efforts. External factors encompass a wide array of data, including economic indicators, weather patterns, social media sentiment, and even geopolitical events. These factors are continually analyzed and synthesized by AI algorithms, resulting in forecasts that are not only more precise but also adaptable to real-time changes.
Imagine an electronics retailer preparing for the holiday season. AI takes into account historical sales data but also factors in emerging tech trends, social media buzz around new gadgets, and the economic health of its target market. This holistic approach ensures that the retailer doesn’t miss out on the latest consumer preferences and can adjust its inventory accordingly.
Case Study: Amazon’s Demand Forecasting Success
Amazon, the undisputed leader in e-commerce, has harnessed the power of AI to refine its demand forecasting. At the heart of this is the recommendation engine, which suggests products to customers based on their browsing and purchase history.
The genius here is twofold. First, it boosts sales by encouraging customers to explore related items. Second, it provides Amazon with invaluable data on customer preferences. This data feeds back into the demand forecasting algorithms, allowing Amazon to fine-tune its predictions continually.
In essence, Amazon’s recommendation engine isn’t just a tool for cross-selling; it’s a dynamic engine that refines its understanding of consumer behavior over time. It’s a testament to how AI-driven demand forecasting can lead to a virtuous cycle of improved accuracy and higher sales.
AI-driven demand forecasting isn’t limited to e-commerce giants like Amazon. Retailers of all sizes and industries are adopting these techniques to gain a competitive edge. It’s a testament to the democratizing power of AI, which is leveling the playing field for businesses in the dynamic world of retail.
Optimizing Stock Levels with AI
Striking the Right Balance
Balancing stock levels is akin to walking a tightrope. Too much inventory ties up capital, increases storage costs, and risks obsolescence. Too little inventory leads to stockouts, disappointed customers, and missed revenue opportunities.
Picture a high-end fashion boutique. Its inventory is a carefully curated collection of designer clothing, each piece representing a substantial investment. If the boutique overstocks and these pieces don’t sell, it could result in significant financial losses. Conversely, if it understocks and customers can’t find what they’re looking for, they’ll take their business elsewhere.
Inventory Turnover: The Key Metric
Inventory turnover is the pulse of retail. It measures how quickly a retailer sells its inventory within a specific period. High turnover suggests that products are in high demand and that capital is flowing efficiently. Low turnover indicates that inventory is stagnating, tying up valuable resources.
AI plays a pivotal role in optimizing inventory turnover. It does so by providing inventory managers with real-time insights into sales trends and stock levels. For instance, during a seasonal promotion, AI might recommend increasing the stock of featured products. Conversely, it might advise reducing inventory of slow-moving items to minimize carrying costs.
Case Study: Walmart’s Inventory Optimization
Walmart, the retail giant known for its vast product assortment, has made substantial investments in AI to optimize inventory. It employs an AI-driven system that continually analyzes historical sales data, supplier information, and even weather forecasts.
Consider the impact of weather on inventory. For a retailer like Walmart, which sells everything from winter coats to swimwear, understanding weather patterns is crucial. If AI predicts a cold front, it can recommend increasing the stock of winter clothing in stores situated in the affected regions. Simultaneously, it can suggest reducing inventory of seasonal items that are unlikely to sell.
Walmart’s AI system also factors in supplier constraints and lead times. If a supplier experiences delays, the system can adjust inventory levels and reorder points accordingly. This agility ensures that customers find what they need on the shelves, enhancing their shopping experience.
In essence, AI doesn’t just optimize inventory; it transforms it into a dynamic and responsive asset. It ensures that products are available when and where customers want them, ultimately driving higher sales and customer satisfaction.
Personalized Marketing with AI
The Power of Personalization
Personalization is the secret sauce of modern marketing. It’s the art of tailoring marketing messages and product recommendations to individual consumers’ preferences and behaviors. Personalized marketing creates a more engaging and relevant shopping experience, and consumers have come to expect it.
Imagine walking into a coffee shop, and the barista knows your name, remembers your favorite latte, and even suggests a new pastry based on your past choices. This level of personalization makes the experience memorable and encourages repeat visits.
Leveraging AI for Personalized Marketing
AI is the driving force behind personalized marketing’s resurgence. It analyzes vast datasets encompassing customer behavior, purchase history, demographic information, and even real-time interactions. From this data, AI can create highly tailored marketing campaigns that resonate with individual customers.
Consider an e-commerce platform that uses AI-driven personalized marketing. When a customer logs in, AI algorithms analyze their past purchases, browsing history, and items in their shopping cart. Based on this data, the platform can recommend products that align with the customer’s preferences. If a customer has a history of buying running shoes, the platform might suggest the latest models or complementary accessories.
AI’s ability to process this data in real-time is its true strength. As a customer browses the website, AI continually adapts its recommendations. If the customer explores outdoor gear, the recommendations shift to hiking boots and camping equipment. This dynamic personalization not only enhances the shopping experience but also drives higher conversion rates.
Case Study: Netflix’s Recommendation Engine
Netflix, the streaming giant, is a prime example of AI-driven personalization. The cornerstone of its platform is the recommendation engine. As you watch shows and movies, Netflix analyzes your viewing habits and user ratings. Based on this data, it suggests content that aligns with your tastes.
Netflix’s recommendation engine is like having a personal movie curator. It understands your genre preferences, knows when you’re in the mood for a thriller or a comedy, and suggests content accordingly. This level of personalization keeps viewers engaged and subscribed.
In essence, AI-powered personalized marketing transforms customers from passive observers to engaged participants. It makes them feel understood and valued, ultimately driving brand loyalty and higher sales.
AI-Powered Supply Chain Management
The Supply Chain Puzzle
The modern supply chain is a sprawling and intricate network that spans continents. It involves the movement of raw materials, components, and finished products, often through multiple intermediaries. Efficient supply chain management is essential for ensuring that products reach stores or customers on time and at the lowest possible cost.
Consider a multinational electronics manufacturer. Its supply chain encompasses suppliers of raw materials, component manufacturers, assembly plants, distribution centers, and retailers. Any disruption or inefficiency within this chain can have far-reaching consequences, from production delays to increased costs.
AI’s Role in Supply Chain Optimization
AI brings a level of sophistication to supply chain management that is unparalleled. It doesn’t just monitor the supply chain; it optimizes it in real-time. Here’s how:
-
Route Optimization: AI can analyze traffic data, weather conditions, and road closures to suggest the most efficient shipping routes. This not only reduces transportation costs but also ensures on-time deliveries.
-
Predictive Maintenance: AI can monitor the condition of equipment and machinery in real-time. When anomalies are detected, it can schedule maintenance before a breakdown occurs. This minimizes downtime and production delays.
-
Inventory Management: AI can optimize inventory levels throughout the supply chain. By analyzing demand patterns, lead times, and supplier reliability, it ensures that products are available when needed without excess inventory tying up capital.
-
Supplier Insights: AI can assess supplier performance and reliability. It can identify potential bottlenecks or risks in the supply chain, allowing companies to proactively address them.
Case Study: Zara’s Agile Supply Chain
Zara, the fashion retailer known for its fast turnaround of styles from the runway to stores, relies heavily on AI in supply chain management. Its success is built on an agile supply chain that responds rapidly to changing fashion trends.
Imagine a scenario where a particular style of dress becomes a social media sensation. It’s featured in a viral TikTok video, and suddenly, everyone wants it. Zara’s AI systems detect this trend in real-time. They analyze social media mentions, online searches, and sales data. Within days, Zara’s production facilities are churning out that dress in high volumes, and it’s in stores while the trend is still hot.
This agility is made possible by AI’s ability to process vast amounts of data quickly. Zara’s supply chain is finely tuned to respond to market demand in near real-time, minimizing the risk of overstocking or missing out on a trend.
In essence, AI in supply chain management transforms it from a static process into a dynamic and responsive system. It ensures that products flow smoothly from manufacturer to consumer, optimizing costs and ensuring timely deliveries.
AI in Pricing Strategies
Dynamic Pricing: A Game-Changer
Pricing in the retail world is not a static affair. It’s a dynamic dance influenced by a multitude of factors such as supply and demand, competitor pricing, and even consumer behavior. Dynamic pricing, the practice of adjusting prices in response to changing market conditions, is where AI shines.
Imagine an airline adjusting ticket prices in real-time based on demand. During peak travel times, prices rise. When demand is low, prices drop. This strategy ensures that airlines maximize revenue while still filling their planes.
Implementing AI-Driven Pricing Strategies
AI analyzes a vast array of data points to inform dynamic pricing decisions. It considers factors such as competitor pricing, historical sales data, real-time demand, and even external events. Let’s delve into how AI makes dynamic pricing a reality:
-
Competitor Analysis: AI continuously monitors competitor pricing, both online and offline. It can even scrape data from competitors’ websites to gain insights. If a competitor lowers their prices, AI can recommend a price adjustment to remain competitive.
-
Demand Sensing: AI algorithms can sense fluctuations in demand and adjust prices accordingly. For example, if a particular toy is trending on social media, AI can detect the surge in interest and recommend a price increase to capitalize on the demand.
-
Real-Time Adjustments: AI enables retailers to make real-time price adjustments. This can be particularly valuable during events like Black Friday or flash sales, where prices need to respond rapidly to market conditions.
Case Study: Uber’s Surge Pricing
Uber’s surge pricing mechanism is a classic example of dynamic pricing driven by AI. During periods of high demand, such as rush hour or inclement weather, Uber’s prices increase. This serves two essential purposes:
-
Incentivizing Drivers: Higher prices attract more drivers to areas with high demand. This ensures that riders can find a ride even during peak times.
-
Managing Demand: By increasing prices, Uber can manage demand more effectively. It discourages riders from taking unnecessary trips and encourages carpooling.
Uber’s surge pricing is a prime example of AI’s ability to respond to real-time market conditions. It’s a win-win; riders can find a ride when they need it, and drivers have the incentive to provide that ride.
In essence, AI in pricing strategies transforms pricing from a static exercise into a dynamic, data-driven science. It ensures that prices reflect market conditions, optimizing revenue and ensuring customers receive value for their money.
The Future of AI in Retail Inventory Management
Emerging Trends and Technologies
As we peer into the future, the role of AI in retail inventory management is poised for remarkable expansion. Several trends and technologies are on the horizon, promising to further revolutionize the retail landscape:
-
Robotic Stock Replenishment: Robots equipped with AI algorithms are becoming more prevalent in distribution centers and even stores. These robots can autonomously restock shelves, reducing labor costs and improving efficiency.
-
Predictive Maintenance: AI will play an increasingly vital role in maintaining store equipment. Sensors will monitor everything from refrigeration units to cash registers, and AI will predict maintenance needs before breakdowns occur.
-
Enhanced Customer Experience: AI-driven virtual shopping assistants will become more sophisticated. These digital helpers will guide customers through stores, provide product recommendations, and answer questions, creating a more engaging shopping experience.
-
AI-Powered Visual Search: Visual search technology, powered by AI, will enable customers to take photos of products they like and find similar items in the store’s inventory. This will bridge the gap between online and offline shopping.
-
Inventory Drones: Drones equipped with AI cameras will be used for inventory management. They can quickly scan shelves and storage areas, ensuring accurate inventory counts.
Preparing for the AI-Driven Future
Retailers must prepare to embrace these emerging trends and technologies to remain competitive. Here’s a roadmap for preparing for the AI-driven future:
-
Invest in AI Infrastructure: Retailers should invest in the necessary hardware and software infrastructure to support AI initiatives. This includes robust data analytics platforms, AI algorithms, and the computational power required for real-time analysis.
-
Data Quality and Security: High-quality data is the lifeblood of AI. Retailers should focus on data collection, quality assurance, and cybersecurity to protect sensitive customer information.
-
Employee Training: Staff should be trained in AI systems’ usage and interpretation. Human oversight remains critical in ensuring AI-driven decisions align with the company’s goals and values.
-
Stay Informed: The AI landscape is continually evolving. Retailers should stay informed about emerging trends, technologies, and best practices to leverage AI effectively.
In essence, the future of AI in retail inventory management holds exciting possibilities. Retailers who embrace these technologies will not only cut costs and boost sales but also deliver enhanced customer experiences that set them apart in the competitive marketplace.
Conclusion
In the dynamic and fiercely competitive world of retail, where margins are often razor-thin, AI marketing stands as a formidable ally in inventory management. From the intricate dance of demand forecasting to the precision of inventory optimization, the personalization of marketing messages to the agility of supply chain management, and the art of dynamic pricing, AI empowers retailers to simultaneously cut costs and boost sales.
As we gaze into the future, AI’s role in retail inventory management is set to expand, offering even more innovative solutions to the challenges faced by retailers. Robotics, predictive maintenance, virtual shopping assistants, visual search, and inventory drones are poised to redefine the retail landscape.
To thrive in this AI-driven future, retailers must invest in AI infrastructure, prioritize data quality and security, train their employees, and stay informed about emerging trends. Those who harness the power of AI will not only survive but also thrive in this ever-evolving retail ecosystem.