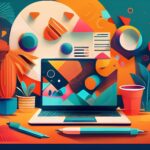
Predictive Marketing Strategies to Skyrocket Your Business in 2024
June 28, 2024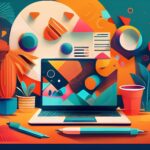
AI-Driven Audience Segmentation for Targeted Media Planning: Boost Your Campaign Performance
June 28, 2024In today’s data-driven world, businesses are constantly seeking ways to stay ahead of the competition and make informed decisions. Predictive analytics has emerged as a powerful tool to achieve this goal. By analyzing historical data and identifying patterns, predictive analytics can forecast future outcomes, helping businesses make proactive and strategic decisions. As we move into 2024, the importance of leveraging predictive analytics for decision-making has never been greater.
Introduction
Predictive analytics combines data mining, machine learning, and statistical techniques to analyze past and current data to make predictions about future events. This technology allows businesses to anticipate trends, understand customer behaviors, and optimize operations. In an era where data is abundant but actionable insights are scarce, predictive analytics stands out as a critical asset for decision-makers.
This article delves into how predictive analytics can enhance decision-making in 2024, exploring various applications, benefits, and best practices for implementation.
1. What is Predictive Analytics?
Predictive analytics involves using statistical algorithms and machine learning techniques to identify the likelihood of future outcomes based on historical data. By analyzing patterns and trends, businesses can make informed predictions that guide decision-making.
Key Concepts
- Data Mining: The process of discovering patterns and relationships in large datasets.
- Machine Learning: Algorithms that enable systems to learn from data and improve over time without explicit programming.
- Statistical Modeling: Techniques used to create models that represent complex relationships within data.
2. The Importance of Predictive Analytics in Business
Predictive analytics is crucial for businesses looking to gain a competitive edge. By leveraging data to anticipate future events, companies can make proactive decisions, mitigate risks, and seize opportunities.
Strategic Planning
Predictive analytics enables businesses to develop strategic plans based on data-driven insights. This approach helps organizations align their goals with market trends and customer needs.
Operational Efficiency
By forecasting demand and optimizing resource allocation, predictive analytics enhances operational efficiency. Businesses can reduce waste, streamline processes, and improve productivity.
Risk Management
Predictive analytics helps identify potential risks and uncertainties. By understanding these factors, businesses can develop strategies to mitigate risks and avoid costly disruptions.
3. Key Applications of Predictive Analytics
Predictive analytics can be applied across various business functions, offering valuable insights and improving decision-making.
Customer Relationship Management (CRM)
Predictive analytics helps businesses understand customer behaviors and preferences, allowing them to tailor marketing campaigns, improve customer service, and increase retention rates.
Supply Chain Management
By predicting demand, supply chain disruptions, and inventory needs, businesses can optimize their supply chain operations, reduce costs, and improve efficiency.
Sales and Marketing
Predictive analytics enables businesses to identify high-potential leads, personalize marketing messages, and optimize sales strategies to increase conversion rates.
Financial Forecasting
Predictive analytics helps businesses forecast revenue, manage budgets, and make informed investment decisions. This approach ensures financial stability and supports long-term growth.
4. Benefits of Predictive Analytics for Decision-Making
The benefits of predictive analytics for decision-making are numerous, providing businesses with a competitive advantage.
Data-Driven Insights
Predictive analytics transforms raw data into actionable insights, enabling businesses to make informed decisions based on facts rather than intuition.
Improved Accuracy
By analyzing historical data and identifying patterns, predictive analytics enhances the accuracy of forecasts and predictions, reducing uncertainty and risk.
Proactive Decision-Making
Predictive analytics allows businesses to anticipate future events and take proactive measures. This approach helps organizations stay ahead of the competition and respond to market changes effectively.
Cost Savings
By optimizing operations and reducing waste, predictive analytics helps businesses save costs. Additionally, accurate forecasts reduce the likelihood of costly mistakes.
5. Essential Tools and Technologies
To effectively implement predictive analytics, businesses need the right tools and technologies.
Data Analytics Platforms
Platforms like IBM Watson, SAS, and Google Cloud AI offer robust predictive analytics capabilities. These tools can handle large datasets, perform complex analyses, and generate accurate predictions.
Machine Learning Frameworks
Frameworks such as TensorFlow, PyTorch, and Scikit-learn provide the necessary infrastructure for developing and deploying machine learning models.
Data Visualization Tools
Tools like Tableau and Power BI help businesses visualize data and insights, making it easier to interpret and act upon the results of predictive analyses.
6. Data Collection and Preparation
Effective predictive analytics begins with collecting and preparing high-quality data.
Data Sources
Gather data from various sources, including transactional data, customer feedback, social media, and IoT devices. This comprehensive dataset provides a holistic view of the business environment.
Data Cleaning
Ensure that the data is accurate, complete, and free of errors. Data cleaning involves removing duplicates, correcting inaccuracies, and addressing missing values.
Data Integration
Integrate data from different sources to create a unified dataset. Use data integration tools and platforms to streamline this process and ensure data consistency.
7. Building Predictive Models
Building accurate predictive models requires expertise in data science and machine learning.
Model Selection
Choose the appropriate predictive model based on the nature of the data and the business problem. Common models include linear regression, decision trees, and neural networks.
Training and Testing
Split the data into training and testing sets. Use the training set to develop the model and the testing set to evaluate its accuracy and performance.
Model Validation
Validate the model using techniques such as cross-validation to ensure it performs well on unseen data. Adjust the model parameters as needed to improve accuracy.
8. Integrating Predictive Analytics into Business Processes
For predictive analytics to be effective, it must be integrated into everyday business processes.
Decision Support Systems
Incorporate predictive analytics into decision support systems to provide real-time insights and recommendations. This integration helps decision-makers act quickly and confidently.
Automated Workflows
Develop automated workflows that use predictive analytics to trigger actions. For example, an e-commerce site might use predictive analytics to recommend products to customers based on their browsing history.
Continuous Monitoring
Regularly monitor the performance of predictive models and update them as needed. Continuous monitoring ensures that the models remain accurate and relevant over time.
9. Case Studies: Success Stories
Learning from real-world examples can illustrate the impact of predictive analytics.
Case Study 1: Walmart’s Inventory Optimization
Walmart uses predictive analytics to optimize its inventory management. By forecasting demand, the company can ensure that products are available when needed, reducing stockouts and excess inventory.
Case Study 2: Netflix’s Content Recommendations
Netflix leverages predictive analytics to recommend shows and movies to its users. This personalized approach keeps users engaged and subscribed to the service.
Case Study 3: Uber’s Surge Pricing
Uber uses predictive analytics to implement surge pricing during peak demand periods. This strategy helps balance supply and demand, ensuring that customers can find rides when needed.
10. Overcoming Challenges in Predictive Analytics
Implementing predictive analytics comes with its own set of challenges.
Data Quality Issues
Ensuring data quality is critical for accurate predictions. Businesses must invest in data cleaning and integration processes to maintain high-quality data.
Complexity of Models
Predictive models can be complex and require specialized knowledge to develop and maintain. Businesses may need to invest in training or hire skilled data scientists.
Resistance to Change
Employees and decision-makers may be resistant to adopting predictive analytics. It’s important to demonstrate the value of predictive analytics and provide training to encourage adoption.
11. Future Trends in Predictive Analytics
As technology evolves, predictive analytics will continue to advance.
AI and Machine Learning Advancements
Continued advancements in AI and machine learning will enhance the capabilities of predictive analytics. Businesses should stay updated with the latest developments and incorporate new technologies.
Real-Time Analytics
Real-time analytics will become increasingly important, allowing businesses to make immediate decisions based on current data.
Integration with IoT
The integration of IoT data with predictive analytics will provide deeper insights into customer behaviors and operational efficiencies.
12. Ethical Considerations
While predictive analytics offers many benefits, it’s important to consider ethical implications.
Data Privacy
Ensure that customer data is collected and used in a way that respects privacy. Comply with data protection regulations and be transparent about data practices.
Bias and Fairness
Be aware of potential biases in predictive models. Take steps to ensure that models are fair and do not discriminate against certain groups.
Transparency
Maintain transparency with customers about how their data is used and how predictive models impact their experiences.
13. Conclusion and Next Steps
Predictive analytics is a powerful tool for enhancing decision-making in 2024. By leveraging data to anticipate future trends and customer behaviors, businesses can make proactive, informed decisions that drive success.
Next Steps
- Evaluate Data Sources: Assess the quality and sources of your data to ensure a solid foundation for predictive analytics.
- Invest in Tools: Adopt the necessary tools and technologies to support predictive analytics efforts.
- Develop Expertise: Build a team with the skills needed to develop and maintain predictive models.
- Integrate Analytics: Incorporate predictive analytics into business processes to make data-driven decisions.
- Monitor and Improve: Continuously monitor the performance of predictive models and make improvements as needed.
By following these steps, businesses can harness the power of predictive analytics to enhance decision-making and achieve their goals in 2024.
FAQs
1. What is predictive analytics?
Predictive analytics uses data mining, machine learning, and statistical techniques to analyze past data and make predictions about future events.
2. How can predictive analytics benefit businesses?
Predictive analytics helps businesses make informed decisions, optimize operations, improve customer relationships, and reduce risks.
3. What are some key tools for predictive analytics?
Essential tools include data analytics platforms (e.g., IBM Watson, SAS), machine learning frameworks (e.g., TensorFlow, PyTorch), and data visualization tools (e.g., Tableau, Power BI).
4. What challenges might businesses face when implementing predictive analytics?
Challenges include ensuring data quality, managing the complexity of predictive models, and overcoming resistance to change within the organization.
5. How can businesses ensure ethical use of predictive analytics?
Businesses should prioritize data privacy, address potential biases in models, and maintain transparency with customers about how their data is used.