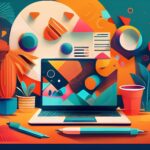
How to Master Keyword Research for Effective SEO
October 23, 2023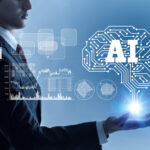
How AI is Transforming the Way Marketers Reach Audiences
October 24, 2023In the rapidly evolving world of marketing, staying ahead of the curve is vital for success. One of the most powerful ways to gain an edge is by harnessing the potential of data analytics in conjunction with AI marketing strategies. This blog post will explore the symbiotic relationship between data analytics and AI marketing, highlighting the advantages and providing practical insights on how to leverage this dynamic duo to supercharge your marketing efforts.
Understanding the Power of Data Analytics in Marketing
The role of data in modern marketing
Data is the cornerstone of modern marketing. In an age where information is abundant, harnessing data’s power is the key to effective marketing strategies. Data encompasses every interaction, transaction, and engagement a customer has with your brand. It’s the digital footprint of your audience, and understanding this data is pivotal.
Data in marketing serves several critical functions:
-
Customer Profiling: It allows you to create detailed customer profiles, including demographics, preferences, and behaviors. By knowing your audience inside out, you can tailor your marketing efforts with pinpoint accuracy.
-
Measuring Success: Data provides the means to track and measure the success of marketing campaigns. You can see which strategies are working and which need adjustment, leading to cost-efficiency and improved ROI.
-
Segmentation: Segmentation is the process of dividing your audience into smaller, more manageable groups. Data-driven segmentation ensures that you’re delivering relevant content to specific groups, increasing the likelihood of conversion.
-
Personalization: The modern customer expects a personalized experience. Data enables you to deliver content and product recommendations that align with individual customer preferences.
Key data analytics techniques for marketers
Data analytics involves a range of techniques, each serving a unique purpose in the marketing realm.
-
Descriptive Analytics: Descriptive analytics deals with the past. It’s about understanding what has happened. By analyzing historical data, you can uncover trends and patterns. For example, it might reveal that a specific product sells better during a particular season. Armed with this knowledge, you can plan marketing campaigns accordingly.
-
Predictive Analytics: Predictive analytics takes things a step further by forecasting future trends. This technique leverages historical data, often with the aid of machine learning algorithms, to predict what’s likely to happen. Imagine a fashion retailer using predictive analytics to foresee which colors will be in vogue next summer. They can stock up on these colors, ensuring they’re ahead of the competition.
-
Prescriptive Analytics: Prescriptive analytics goes beyond predicting outcomes; it offers suggestions for optimal actions. It’s like having a marketing consultant at your fingertips. For instance, if you run an e-commerce store, prescriptive analytics can recommend the best time to run a flash sale based on historical data and current market conditions.
Case studies of data-driven marketing successes
Let’s explore a few real-world examples of how data-driven marketing has transformed businesses.
-
Amazon’s Personalized Recommendations: Amazon’s success isn’t solely due to its vast inventory but also its data-driven recommendation engine. By analyzing customer purchase history, browsing behavior, and even what’s in their shopping carts, Amazon suggests products relevant to each user. This approach has significantly increased sales and customer engagement. In fact, around 35% of Amazon’s revenue comes from these personalized recommendations.
-
Netflix’s Content Customization: Netflix’s data analytics prowess is legendary. It doesn’t just stop at recommending what movie to watch next; it even influences content creation. By analyzing what viewers like to watch, Netflix has produced hit series like “House of Cards” and “Stranger Things.” They knew these shows would resonate with their audience even before a single episode was shot.
-
IBM Watson’s Marketing Success: IBM Watson, an AI-powered platform, is helping businesses harness data effectively. Watson offers insights into customer behavior, market trends, and competitor strategies. For example, The North Face, a leading outdoor apparel brand, used Watson to enhance its customer service by providing personalized product recommendations. This led to a 60% increase in online sales and a 35% increase in click-through rates.
Common data analytics challenges
While data analytics is a game-changer, it’s not without its challenges.
-
Data Quality: Garbage in, garbage out. If your data is inaccurate or incomplete, your analyses will be flawed. Ensuring data quality is a constant challenge. This includes cleaning, validating, and maintaining data integrity.
-
Data Privacy: In an era of data breaches and privacy concerns, handling customer data ethically and securely is paramount. Compliance with regulations like GDPR and CCPA is a must.
-
Data Integration: Companies often have data stored in various silos. Integrating this data to create a unified view can be complex and time-consuming. However, the insights gained from such integration are invaluable.
-
Talent Shortage: Skilled data analysts are in high demand. Finding and retaining talented professionals can be a challenge for businesses. It’s a competitive landscape where data scientists and analysts are like gold dust.
In the fast-evolving marketing landscape, understanding and navigating these challenges while leveraging data analytics is crucial for success.
Unraveling the Magic of AI in Marketing
What is AI marketing?
AI marketing is the epitome of marketing in the digital age. It involves the application of artificial intelligence to enhance marketing strategies and campaigns. At its core, AI marketing leverages advanced algorithms and computational power to make sense of the vast amount of data generated by online activities.
AI marketing is not limited to a single technology; it encompasses various AI branches, including:
-
Machine Learning: This is perhaps the most prevalent AI technology in marketing. Machine learning algorithms analyze data to identify patterns, make predictions, and adapt strategies.
-
Natural Language Processing (NLP): NLP enables AI systems to understand and generate human language. Chatbots, sentiment analysis, and content creation are some NLP applications in marketing.
-
Computer Vision: For businesses dealing with visual content, like e-commerce, computer vision is invaluable. It allows for the automatic tagging and categorization of images and videos.
-
Predictive Analytics: Predictive analytics, powered by AI, helps businesses foresee trends, customer behavior, and even potential issues.
Benefits of AI in marketing
AI marketing offers a plethora of advantages, making it a must-have for businesses striving to thrive in the digital era.
-
Enhanced Personalization: AI can analyze vast amounts of data to create highly personalized content, product recommendations, and marketing messages. This personalization leads to increased customer engagement and higher conversion rates.
-
Efficiency: Automation is at the heart of AI marketing. It can handle repetitive tasks, such as sending follow-up emails, managing social media posts, and analyzing customer behavior. This automation frees up marketers to focus on strategy, creativity, and high-value tasks.
-
Real-time Insights: AI can provide real-time data analysis, helping marketers stay on top of changing trends and customer behavior. Instead of relying on historical data, you can respond proactively to emerging opportunities and threats.
-
Predictive Analytics: Predictive analytics is a natural fit for AI. By analyzing vast datasets and identifying patterns, AI can predict future customer behavior and trends. This predictive power enables marketers to plan and execute campaigns with foresight.
AI-driven personalization in marketing
Personalization in marketing is not a new concept. However, AI takes it to a whole new level. Here’s how AI-driven personalization works:
-
Data Collection: AI gathers data from various touchpoints, including website visits, social media interactions, and purchase history. This data forms the foundation for personalization.
-
Pattern Recognition: AI algorithms recognize patterns and trends within the data. For instance, it might identify that customers who purchase a particular product are more likely to engage with certain types of content.
-
Content Customization: Based on the patterns detected, AI customizes content and recommendations for individual customers. For example, if a customer frequently buys running shoes, AI might recommend related products or blog posts on running tips.
-
Real-time Adaptation: AI is not static. It adapts in real-time as customer behavior evolves. If a customer’s preferences change, the AI system adjusts its recommendations accordingly.
The result is a highly personalized experience that resonates with customers and drives engagement. It’s like having a personal shopper or concierge service, tailored to each customer’s needs.
Real-world applications of AI marketing
AI marketing isn’t just a theoretical concept; it’s a practical and proven approach that businesses across various industries are adopting. Let’s explore a few real-world applications:
-
Chatbots: AI-powered chatbots have revolutionized customer support. They can answer inquiries 24/7, provide instant responses, and even engage in natural conversations. This not only enhances customer satisfaction but also reduces the workload on human support agents.
-
Email Marketing: Email marketing is a powerful tool for customer engagement. AI can take it to the next level by analyzing customer behavior to send highly personalized email recommendations. For example, if a customer frequently buys books on history, an AI-driven email might recommend a new release in historical fiction.
-
Dynamic Pricing: AI-driven dynamic pricing is a game-changer for e-commerce and travel industries. These algorithms analyze market conditions, competitor pricing, and customer behavior to adjust prices in real-time. The result is optimized revenue and a competitive edge.
These applications showcase the versatility of AI in marketing, from improving customer service to fine-tuning pricing strategies. The common thread is the ability to leverage data for better decision-making, efficiency, and personalization.
Now that we’ve explored the role of data analytics and AI in marketing, it’s time to see how they come together in a powerful synergy.
Where Data Analytics Meets AI in Marketing
How data analytics and AI complement each other
Data analytics and AI are like two sides of the same coin in marketing. Data analytics provides the raw material, and AI processes and interprets that data to generate actionable insights. It’s a symbiotic relationship where data analytics feeds AI, and AI enhances the capabilities of data analytics.
Here’s how they complement each other:
-
Data Enrichment: Data analytics enriches AI models by providing historical data. Imagine you’re launching a new product, and you want to predict its potential success. Data analytics can provide historical sales data, customer behavior, and market trends. AI models can then use this data to make predictions.
-
Real-time Insights: While data analytics is great for historical analysis, AI excels in real-time data processing. When a customer visits your website and interacts with it, AI can instantly analyze their behavior and provide real-time recommendations. This level of responsiveness is difficult to achieve with traditional data analytics alone.
-
Predictive Power: AI’s strength lies in predictive analytics. By examining vast datasets, it can predict future trends and customer behavior. Data analytics sets the stage by offering the data for AI to work its magic. This synergy results in highly accurate predictions.
-
Automation: AI automates various marketing processes, from content generation to social media posting. Data analytics is crucial in feeding these AI systems with the right data to ensure they make informed decisions.
Data-driven insights for AI marketing
Data analytics is the starting point for AI marketing. It provides the insights and data needed for AI models to make intelligent decisions. Here’s how data analytics fuels AI marketing:
-
Customer Behavior Analysis: Data analytics can reveal patterns in customer behavior. For instance, it might show that customers who buy outdoor equipment are more likely to purchase camping gear in the spring. AI models can then use this data to tailor marketing messages and product recommendations during that season.
-
Segmentation: Data analytics helps in segmenting customers into different groups based on their behavior and preferences. For example, a clothing retailer might segment customers into “casual wear” and “formal wear” shoppers. AI can then target these segments with specific product recommendations and content.
-
Content Optimization: Data analytics can identify the type of content that resonates most with your audience. AI can then use this information to generate similar content or recommend it to relevant customers.
-
Sales Forecasting: Predicting future sales is a vital part of marketing. Data analytics provides historical sales data, which AI can analyze to create highly accurate sales forecasts. This is invaluable for inventory management and campaign planning.
AI-enhanced data analytics tools
The marriage of AI and data analytics has given rise to a new generation of tools that empower marketers to extract deeper insights from their data.
-
Customer Segmentation Tools: AI-driven customer segmentation tools automatically categorize customers based on their behavior, demographics, and preferences. This allows for highly targeted marketing campaigns.
-
Sentiment Analysis Tools: AI is exceptional at analyzing sentiment in text data. Sentiment analysis tools can gauge public sentiment about your brand or products on social media, forums, and reviews. This helps in reputation management and understanding customer feelings.
-
Anomaly Detection Tools: AI can detect unusual patterns or behaviors within your data. For instance, it can identify a sudden surge in website traffic or an unexpected drop in sales. This information allows marketers to respond swiftly to opportunities or issues.
-
Personalization Engines: AI-driven personalization engines use data analytics to create personalized experiences for customers. These engines can analyze a customer’s past interactions with your brand and tailor content and product recommendations accordingly.
This new generation of tools saves time, automates complex processes, and ensures data-driven decision-making.
Creating a synergy between AI and data analytics
To fully harness the power of the synergy between AI and data analytics, businesses should consider a few key strategies:
-
Integrate Data Sources: Ensure that data from various sources within your organization is integrated and accessible to both data analytics and AI systems. A unified data source enhances the accuracy and completeness of insights.
-
Real-time Data Analysis: Combine data analytics with real-time AI systems to enable instant decision-making. For example, if a customer abandons their shopping cart, an AI system can send a personalized offer to entice them to complete the purchase.
-
Feedback Loop: Create a feedback loop where insights generated by AI are used to refine data analytics techniques. For instance, if AI discovers that a specific product recommendation strategy is highly effective, data analytics can delve deeper into the data to understand why.
-
Training AI Models: Data analytics can be invaluable in training AI models. It can provide the labeled data needed for supervised learning. For example, data analytics can label customer interactions as positive, negative, or neutral, which AI models can then use to understand sentiment.
By implementing these strategies, businesses can fully capitalize on the complementary strengths of data analytics and AI in marketing. This synergy enables smarter decision-making, real-time personalization, and more efficient marketing campaigns.
Practical Implementation of Data Analytics in AI Marketing
Data collection and storage for AI-driven insights
The implementation of data analytics in AI marketing begins with robust data collection and storage. Here are some strategies to consider:
-
Utilizing CRM Systems: Customer Relationship Management (CRM) systems are a goldmine of customer data. They provide a centralized repository for customer interactions, purchase history, and preferences. By integrating AI with CRM systems, businesses can extract valuable insights to fuel marketing strategies.
-
Data Warehouses: Storing data in dedicated data warehouses is a best practice. These warehouses allow for centralized and easy access to data from various sources. They are designed to handle large datasets efficiently, making them ideal for AI-driven analysis.
-
IoT Data: The Internet of Things (IoT) is a growing source of data. Smart devices, sensors, and wearables generate a wealth of information. In sectors like healthcare and retail, IoT data can provide valuable insights. For example, a fitness tracker can provide data on user activity and health metrics, which can be used to personalize fitness-related marketing campaigns.
-
API Integrations: Application Programming Interfaces (APIs) allow businesses to tap into external data sources. For instance, weather data can influence marketing strategies for businesses in industries like agriculture, fashion, or travel.
-
Data Cleaning and Validation: Data quality is paramount. Before data can be useful for AI marketing, it needs to be cleaned and validated. This process involves removing duplicates, correcting errors, and ensuring data consistency. Data that is inconsistent or riddled with errors can lead to inaccurate AI predictions and decisions.
Data preprocessing for AI models
Data preprocessing is a crucial step in AI marketing. Before AI models can work their magic, data needs to be transformed and prepared. Here are some data preprocessing techniques:
-
Data Normalization: Normalizing data means scaling it to a standard range. For example, if you’re analyzing customer spending, you might normalize the data to a range between 0 and 1. This ensures that variables with different units or scales are treated equally by AI models.
-
Feature Engineering: Feature engineering involves creating new features from existing data. For instance, you might create a “customer engagement score” based on various factors like website visits, email opens, and social media interactions. These engineered features provide more context for AI models.
-
Data Imputation: Incomplete data is a common issue. Data imputation techniques fill in missing data points with estimated values. For example, if you have a customer record with missing purchase history, imputation methods can estimate the missing values based on similar customers’ behavior.
-
Outlier Detection: Outliers are data points that deviate significantly from the norm. AI models can be sensitive to outliers. Detecting and handling outliers is essential to prevent them from skewing AI predictions.
-
Text and Image Processing: If your data includes text or images, preprocessing is required to make these types of data understandable to AI models. Natural Language Processing (NLP) techniques can be used to analyze text, while computer vision techniques handle images.
Data preprocessing is a meticulous process that ensures data is in the right format and quality for AI models to work effectively. It’s the bridge between raw data and actionable insights.
Leveraging predictive analytics with AI
Predictive analytics is a cornerstone of AI marketing. It involves using historical data and machine learning algorithms to make predictions about future customer behavior and trends. Here’s how to leverage predictive analytics with AI:
-
Selecting the Right Algorithm: There are various machine learning algorithms for predictive analytics, such as linear regression, decision trees, and neural networks. The choice of algorithm depends on the type of data and the nature of the prediction task.
-
Training the Model: To make predictions, AI models need to be trained on historical data. For instance, if you want to predict customer churn, the model needs to learn from past instances of customers who churned and those who didn’t.
-
Feature Selection: Not all data features are relevant for prediction. Feature selection helps identify the most important variables that impact the prediction. For instance, when predicting website traffic, factors like time of day, season, and advertising spend can be critical.
-
Validation and Testing: Predictive models should be validated and tested to ensure accuracy. This involves splitting data into training and testing sets and measuring the model’s performance against known outcomes.
-
Deploying Predictive Models: Once a predictive model is trained and validated, it can be deployed in real-world scenarios. For instance, it can be used to predict which products a customer is likely to purchase and offer those recommendations in real-time.
Predictive analytics with AI is a dynamic process that constantly learns and evolves as new data becomes available. It’s a powerful tool for businesses to anticipate customer needs and behavior.
Measuring the success of AI marketing campaigns with data
The success of AI marketing campaigns hinges on the ability to measure their impact. Data analytics plays a crucial role in this process. Here’s how to measure the success of AI marketing campaigns:
-
Key Performance Indicators (KPIs): Define clear KPIs for your campaigns. These could include metrics like conversion rate, click-through rate, customer acquisition cost, and customer lifetime value.
-
A/B Testing: Conduct A/B tests to compare the performance of AI-driven campaigns against traditional campaigns. This helps in quantifying the impact of AI.
-
Attribution Modeling: Use attribution models to understand the customer journey and identify touchpoints that contribute to conversions. This can reveal the influence of AI-driven personalization on customer decisions.
-
Customer Feedback: Collect customer feedback to gauge satisfaction with AI-driven experiences. Surveys, reviews, and direct customer feedback are valuable sources of information.
-
Advanced Analytics: Implement advanced analytics techniques to gain deeper insights into campaign performance. For example, marketing mix modeling can help determine the impact of AI marketing on overall revenue.
-
ROI Calculation: Calculate the return on investment (ROI) for AI marketing campaigns. This involves comparing the costs of AI implementation against the additional revenue generated due to AI-driven personalization and recommendations.
-
Continuous Improvement: Use data analytics to identify areas for improvement. AI marketing is an evolving field, and constant refinement is essential. For example, if certain product recommendations aren’t performing well, data analytics can reveal this and suggest adjustments.
By using data analytics to measure the success of AI marketing campaigns, businesses can make data-driven decisions on campaign optimization, budget allocation, and strategic adjustments.
Incorporating data analytics into AI marketing is more than a trend; it’s a necessity. It’s the bridge that connects raw data to actionable insights, making AI marketing strategies effective and efficient.
Now, let’s explore some real-world examples of successful integration of data analytics and AI in marketing.
Case Studies: Successful Integration of Data Analytics and AI in Marketing
The synergy between data analytics and AI has led to remarkable success stories in marketing. Let’s delve into some inspiring case studies to see how businesses have leveraged this fusion to achieve outstanding results.
Amazon’s Recommendation Engine: A Case Study in Data-Driven Personalization
Amazon, the e-commerce giant, is renowned for its data-driven approach to marketing. At the heart of its marketing strategy is the recommendation engine, powered by a fusion of data analytics and AI. This engine is responsible for those personalized product recommendations you see every time you visit the Amazon website.
The Power of Data Collection: Amazon collects an immense amount of data from its customers. This includes purchase history, browsing behavior, items added to the shopping cart, and even items left in the cart without purchase. The data analytics team at Amazon uses this vast dataset to gain insights into customer preferences and behaviors.
Machine Learning Algorithms: AI, in the form of machine learning algorithms, takes over to analyze this data. These algorithms examine patterns in customer behavior, such as which products are frequently bought together, what’s commonly viewed, and what’s abandoned in shopping carts. The result is a finely-tuned recommendation system.
Highly Personalized Recommendations: The AI-driven recommendation engine uses the insights from data analytics to offer highly personalized recommendations to customers. For example, if you recently bought a camera, the engine might suggest complementary items like a tripod, memory card, or camera bag.
Continuous Learning: The beauty of this system is that it continuously learns. As you interact with the platform, it adapts to your changing preferences and behavior, ensuring that the recommendations remain relevant.
Staggering Impact: The impact of this personalized approach is staggering. It’s estimated that around 35% of Amazon’s revenue comes from these personalized recommendations. Customers are more likely to make a purchase when presented with products that align with their interests and needs.
The Amazon case study demonstrates the potent fusion of data analytics for insights and AI for personalization, resulting in a marketing strategy that keeps customers engaged and boosts revenue.
Netflix’s Dynamic Content Optimization through AI and Data Analytics
Netflix is a trailblazer in the entertainment industry, and its use of AI and data analytics in content recommendation and creation is a game-changer.
Data Collection and Analysis: Netflix collects data on what its subscribers watch, how long they watch, when they pause or rewind, and even what they search for. Data analytics examines this wealth of information to identify viewer preferences and trends.
Personalized Content: AI algorithms then kick in to create personalized content recommendations. These recommendations aren’t just for what to watch next but also influence content creation. For instance, when producing a series like “House of Cards,” Netflix knew it would be a hit based on data insights about subscriber preferences for political dramas and actor Kevin Spacey.
Content Testing: Netflix tests various elements of a show, such as artwork, trailers, and descriptions, to see what captures viewers’ attention. These tests are crucial for fine-tuning how content is presented to subscribers.
Global Insights: Data analytics and AI enable Netflix to understand what viewers around the world want. This influences decisions on the acquisition of international content and the creation of local original content tailored to different regions.
The result is a streaming platform that offers a highly personalized experience, keeping subscribers engaged and reducing churn. The data-driven approach extends to content creation, ensuring that Netflix produces shows that resonate with its global audience.
IBM Watson’s AI-Driven Marketing Success
IBM Watson, a powerful AI platform, is a prime example of how data analytics and AI are transforming marketing.
Data Insights with Watson: Watson helps businesses derive insights from massive datasets. In the case of marketing, it analyzes customer behavior, market trends, and competitor strategies to provide valuable insights.
Personalization and Recommendations: Watson offers a high degree of personalization in marketing. It can analyze a customer’s past interactions with a brand to provide personalized product recommendations.
Conversational Marketing: Watson is also behind IBM’s efforts in conversational marketing. It powers chatbots and virtual assistants that can engage with customers in natural language, answer inquiries, and provide a seamless customer experience.
Real-Time Analysis: Watson provides real-time data analysis, enabling businesses to respond swiftly to changing market conditions and customer behavior.
IBM Watson’s success stories are varied, spanning industries from healthcare to finance. For example, The North Face, a prominent outdoor apparel brand, used Watson to enhance its customer service. Watson provided personalized product recommendations to online shoppers, resulting in a 60% increase in online sales and a 35% increase in click-through rates.
These case studies illustrate how the fusion of data analytics and AI is propelling businesses to new heights in marketing. Whether it’s e-commerce, entertainment, or technology, the combination of data-driven insights and AI-driven personalization is a winning strategy.
Challenges and Ethical Considerations in Data-Driven AI Marketing
While the marriage of data analytics and AI in marketing offers incredible potential, it’s not without its challenges and ethical considerations.
Data Privacy and Consumer Concerns
With data being the lifeblood of data-driven marketing, the issue of data privacy is paramount. Consumers are increasingly concerned about how their data is collected, used, and shared. The misuse of customer data can lead to significant reputational damage and legal consequences.
To navigate this challenge, businesses must:
-
Transparency: Be transparent about data collection and usage. Customers should know what data is being collected, how it will be used, and if it will be shared with third parties.
-
Consent: Obtain clear and informed consent from customers before collecting and using their data. This means giving customers the choice to opt in or opt out.
-
Data Security: Implement robust data security measures to protect customer data from breaches and unauthorized access.
-
Compliance: Stay updated with data privacy regulations, such as GDPR, CCPA, and others, and ensure compliance with these laws.
Algorithmic Bias and Fairness in AI Marketing
AI systems, including those used in marketing, are susceptible to bias. Bias can emerge from biased training data or the design of algorithms. This bias can result in unfair treatment, discrimination, and unequal opportunities for certain groups of people.
To mitigate algorithmic bias:
-
Diverse Training Data: Ensure training data is diverse and representative of different demographics and backgrounds.
-
Bias Audits: Regularly conduct audits to identify and rectify bias in algorithms.
-
Explainability: Make AI systems more explainable, so it’s clear why a particular recommendation or decision was made.
-
Ethical AI Design: Design AI systems with fairness, accountability, and transparency in mind from the outset.
Compliance with Data Regulations
The ever-evolving landscape of data regulations poses a challenge for businesses. Staying compliant with various regional and international data laws can be complex.
To address this challenge:
-
Legal Expertise: Employ legal experts or consultants who specialize in data privacy and compliance to navigate the legal complexities.
-
Data Governance: Establish strong data governance practices to ensure data is handled in accordance with regulations.
-
Consent Management Platforms: Use consent management platforms to streamline compliance with data regulations and maintain records of customer consent.
-
Cross-Border Data Transfer: Be aware of cross-border data transfer regulations, especially when handling data from customers in different countries.
Mitigating Risks in Data Analytics and AI Marketing
The fast-paced nature of data analytics and AI marketing can introduce risks. It’s crucial for businesses to take proactive steps to mitigate these risks.
-
Testing and Validation: Rigorously test AI algorithms and data analytics models to ensure accuracy and reliability.
-
Human Oversight: Maintain human oversight of AI systems to catch errors and biases.
-
Educating Staff: Educate marketing and data analytics teams about data privacy and ethical considerations to ensure everyone is on the same page.
-
Crisis Management: Develop crisis management plans in case of data breaches or ethical dilemmas, and be ready to respond quickly.
By acknowledging these challenges and ethical considerations and taking proactive steps to address them, businesses can leverage data analytics and AI in marketing responsibly and effectively.
The Future of Data Analytics and AI in Marketing
The future of data analytics and AI in marketing is filled with exciting possibilities. Here are some key trends and developments to watch for:
Hyper-Personalization
AI-driven hyper-personalization will become even more precise. AI systems will analyze data on an individual level, offering recommendations and content tailored to each person’s unique preferences and behavior.
Voice and Visual Search
Voice and visual search will continue to gain prominence. AI-driven search systems will recognize and understand spoken and visual queries, making it easier for consumers to find products and information.
AI-Generated Content
AI will play a greater role in content generation. From writing product descriptions to creating marketing copy, AI algorithms will produce content that’s indistinguishable from human-generated text.
Predictive Analytics Evolution
Predictive analytics will become more accurate and sophisticated. AI will have a greater role in forecasting trends and customer behavior, helping businesses stay ahead of the curve.
Ethical AI and Transparency
Ethical AI will be a top priority. Businesses will need to be transparent about how AI is used, and ethical considerations will be at the forefront of AI system design.
Augmented Reality (AR) Marketing
AR will play a more significant role in marketing. AI-driven AR experiences will allow consumers to interact with products in immersive and interactive ways.
Integration of Data from IoT
With the growth of the Internet of Things (IoT), data from smart devices will be integrated into marketing strategies. This will provide a wealth of data for personalization and targeting.
Data-Driven Storytelling
Storytelling in marketing will be data-driven. AI will analyze customer data to create compelling narratives that resonate with audiences.
As data analytics and AI continue to evolve, businesses that embrace these trends will have a competitive edge in the marketing landscape of the future.
Conclusion
The integration of data analytics and AI has revolutionized marketing. This dynamic duo empowers businesses to understand their customers on a profound level, offer highly personalized experiences, and make data-driven decisions. The case studies we’ve explored demonstrate the tangible benefits of this synergy, from Amazon’s personalized recommendations to Netflix’s content customization and IBM Watson’s marketing success.
However, this fusion is not without challenges, including data privacy, algorithmic bias, and the need for compliance with data regulations. Businesses must navigate these challenges to ensure responsible and effective data-driven AI marketing.
The future of data analytics and AI in marketing promises even more exciting developments, from hyper-personalization and AI-generated content to ethical AI and augmented reality marketing. To stay competitive, businesses should embrace these trends and leverage the power of data analytics and AI in their marketing strategies.
In this ever-evolving marketing landscape, one thing is clear: the future belongs to those who understand the transformative potential of data analytics and AI in marketing. It’s not just about selling products; it’s about building meaningful and personalized relationships with customers, and this synergy is the key to achieving that goal. So, let data be your guide, and let AI be your marketing companion in this exciting journey towards success.