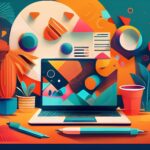
Mastering the Art of Ad Creative Design: Revolutionizing Marketing with AI
September 25, 2023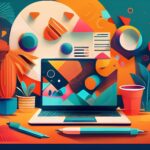
Cultivating Seamless Customer Support in the Age of AI Marketing
September 27, 2023E-commerce has evolved at an astonishing pace, and at its core lies the digital transformation powered by Artificial Intelligence (AI). In this section, we’ll delve into the fascinating journey of e-commerce, the pivotal role of AI, and the profound impact of recommendation engines.
A. The Evolution of E-commerce
The roots of e-commerce can be traced back to the early days of the internet when businesses first recognized the potential of online transactions. In the late 1990s and early 2000s, e-commerce was synonymous with clunky websites and limited product offerings. But as technology advanced, so did online shopping.
Today, e-commerce has transcended its humble beginnings. It’s a global phenomenon, allowing consumers to browse and purchase products from anywhere, at any time. From the convenience of mobile shopping apps to the seamless checkout experiences, e-commerce has become an integral part of our lives.
B. The Role of AI in E-commerce
Enter Artificial Intelligence—a technological marvel that’s redefining the e-commerce landscape. AI algorithms, machine learning, and data analytics are the driving forces behind this transformation. They enable e-commerce platforms to understand, predict, and respond to consumer behavior like never before.
AI enhances product recommendations, optimizes supply chains, and personalizes marketing strategies. It’s the reason why you see product suggestions tailored to your preferences or receive emails with offers that seem custom-made for you. In essence, AI is the wizard behind the digital storefront curtain, making e-commerce more efficient and user-centric.
C. Significance of Recommendation Engines
One of the stars of this AI-driven show is recommendation engines. These intelligent systems have revolutionized how consumers discover products and services. They analyze vast amounts of data to suggest items that align with a user’s interests, buying history, and even real-time behavior.
The significance of recommendation engines cannot be overstated. They boost sales by increasing product visibility, enhance user engagement, and foster customer loyalty. Think about Amazon’s “Customers who bought this also bought” section or Netflix’s personalized movie recommendations. Recommendation engines have become an integral part of the e-commerce experience, shaping purchasing decisions and user satisfaction.
Understanding AI Marketing
In this section, we’ll unravel the mysteries of AI marketing. What exactly is it, and why is it a game-changer in the world of e-commerce? Let’s embark on this journey of discovery.
A. What is AI Marketing?
AI marketing, also known as marketing automation, represents the fusion of artificial intelligence and marketing strategies. It involves using AI technologies to streamline and optimize various marketing tasks. The goal is to make marketing efforts more efficient, data-driven, and personalized.
AI marketing encompasses a wide range of applications, from email automation and chatbots to predictive analytics and customer segmentation. It empowers businesses to understand their customers better, deliver tailored messages, and ultimately, drive higher conversion rates.
B. Benefits of AI Marketing in E-commerce
Now, let’s dive into the myriad benefits AI marketing brings to the e-commerce table. Each of these advantages plays a pivotal role in enhancing the customer experience and boosting a company’s bottom line.
1. Personalization
Imagine walking into a physical store, and the salesperson already knows your preferences, style, and budget. AI marketing replicates this personalized experience online. It analyzes user data and behavior to recommend products that align with a customer’s tastes, previous purchases, and browsing history.
This level of personalization not only improves customer satisfaction but also increases the likelihood of conversions. When customers feel understood and catered to, they’re more likely to make a purchase.
2. Predictive Analytics
AI has the remarkable ability to predict future trends and consumer behavior. By analyzing historical data, AI can forecast what products are likely to be in demand, which marketing strategies will be effective, and when customers are most likely to make a purchase.
This predictive prowess empowers e-commerce businesses to stay one step ahead of the competition. They can proactively adjust their product offerings, marketing campaigns, and pricing strategies based on AI-generated insights.
3. Customer Segmentation
Not all customers are the same, and treating them as such can lead to missed opportunities. AI marketing excels at segmenting customers based on their behavior, demographics, and preferences.
Segmentation allows businesses to create targeted marketing campaigns. For example, a clothing retailer can send different promotions to customers interested in athletic wear versus those who prefer formal attire. This precision increases the relevance of marketing efforts and drives higher engagement rates.
4. Dynamic Pricing
Have you ever noticed that the price of a product on an e-commerce website can change from one day to the next? This dynamic pricing strategy is another gift from AI marketing.
Dynamic pricing algorithms analyze market conditions, competitor prices, and customer demand to adjust product prices in real-time. For example, during a high-demand period, a hotel might increase its room rates, while a fashion retailer could offer discounts on winter coats as summer approaches.
Dynamic pricing ensures that prices remain competitive and aligned with market dynamics, maximizing revenue while keeping customers satisfied.
C. AI Marketing Tools and Technologies
The world of AI marketing is brimming with tools and technologies designed to simplify complex tasks and deliver tangible results. Let’s take a quick tour of some of these remarkable resources:
-
Chatbots: AI-powered chatbots provide instant customer support, answer queries, and guide users through the purchase process. They operate 24/7, enhancing user satisfaction and reducing response times.
-
Email Automation: Email marketing is a powerful tool, and AI makes it even more effective. Automated email campaigns can be personalized based on user behavior, ensuring that subscribers receive content and offers that resonate with their interests.
-
Predictive Analytics Platforms: These platforms use AI to analyze vast datasets and generate insights. They can predict customer behavior, recommend marketing strategies, and even forecast sales trends.
-
Content Recommendation Engines: Much like product recommendation engines, content recommendation engines suggest articles, blog posts, or videos based on a user’s preferences. This keeps users engaged and encourages them to explore more content.
-
Social Media Management Tools: AI-driven social media tools can schedule posts, analyze engagement, and even suggest content ideas based on trending topics and user interactions.
These tools and technologies are the backbone of AI marketing, making it accessible and effective for businesses of all sizes.
The Magic of Recommendation Engines
In this section, we’ll demystify recommendation engines. How do they work, what are the different types, and why are they indispensable in the world of e-commerce? Let’s explore the enchanting world of recommendation engines.
A. How Recommendation Engines Work
At the core of recommendation engines lies intricate algorithms that sift through mountains of data to provide users with personalized recommendations. Here’s a simplified breakdown of how these engines work their magic:
-
Data Collection: Recommendation engines start by collecting data about users and their interactions with the platform. This data includes browsing history, search queries, purchases, and even user ratings and reviews.
-
Data Preprocessing: Once the data is collected, it goes through preprocessing, where noise is removed, and relevant features are extracted. This step ensures that the data is clean and ready for analysis.
-
Feature Engineering: Features or attributes of products and users are identified and defined. For instance, in e-commerce, product features might include category, price, brand, and user features could include age, location, and browsing behavior.
-
Algorithm Selection: Recommendation engines use various algorithms to analyze user and product data. These algorithms include collaborative filtering, content-based filtering, and hybrid systems, each with its unique approach.
-
Generating Recommendations: After analyzing the data, the engine generates a list of recommendations for each user. These recommendations are based on patterns and similarities found in the data.
-
Feedback Loop: As users interact with the recommendations (e.g., by making purchases or providing feedback), the engine continues to learn and refine its suggestions. This feedback loop is essential for improving the accuracy of recommendations over time.
-
Presentation to Users: Finally, the recommendations are presented to users through various channels, such as the website’s homepage, product pages, or personalized emails.
This process, powered by AI and machine learning, happens in the background, creating a seamless and personalized shopping experience for users.
B. Types of Recommendation Engines
Not all recommendation engines are created equal. They come in different flavors, each suited to specific use cases. Let’s explore the three primary types of recommendation engines:
1. Collaborative Filtering
Collaborative filtering relies on the principle of “users who liked this also liked.” It identifies users who have similar preferences and recommends products that those similar users have enjoyed.
For example, if User A and User B have both purchased and liked similar books, collaborative filtering would recommend books that User A hasn’t seen but that User B has enjoyed.
Collaborative filtering can be further categorized into user-based and item-based approaches, each with its advantages and drawbacks.
2. Content-Based Filtering
Content-based filtering, on the other hand, focuses on the attributes of products and users. It recommends items based on their similarity to what a user has interacted with in the past.
For instance, if a user has shown interest in science fiction books, a content-based recommendation engine would suggest other science fiction titles, considering attributes such as genre, author, and plot.
Content-based filtering is excellent for diversifying recommendations and can work well for niche markets.
3. Hybrid Recommendation Systems
Sometimes, one approach isn’t enough to provide highly accurate recommendations. Hybrid recommendation systems combine elements of collaborative filtering and content-based filtering to deliver more precise suggestions.
These systems take advantage of the strengths of both methods. They may use collaborative filtering to identify user preferences and then refine recommendations with content-based filtering to ensure relevance.
Hybrid systems are versatile and can adapt to various e-commerce scenarios, making them a popular choice for many platforms.
C. Success Stories: Companies Leveraging Recommendation Engines
The proof of the pudding is in the eating, and recommendation engines have served some of the world’s biggest e-commerce giants well. Let’s explore a few success stories to understand the real-world impact of these engines:
-
Amazon: The online retail behemoth is a prime example of recommendation engine mastery. Its “Customers who bought this also bought” and “Frequently bought together” sections are driven by sophisticated recommendation algorithms. These features significantly contribute to Amazon’s remarkable conversion rates and revenue.
-
Netflix: When you think of personalized content recommendations, Netflix immediately comes to mind. Its recommendation engine analyzes viewing history, user ratings, and even the time spent browsing to serve up movie and TV show suggestions that keep subscribers engaged.
-
Spotify: Music streaming service Spotify uses recommendation engines to curate playlists tailored to users’ music preferences. The “Discover Weekly” playlist, which is automatically updated each week, is a hit among users and keeps them coming back for more.
These success stories illustrate how recommendation engines have become integral to the e-commerce experience, driving engagement, retention, and revenue for businesses across industries. They’re not just a fancy feature; they’re a competitive advantage.
Challenges and Ethical Considerations
As powerful as recommendation engines are, they are not without their challenges and ethical considerations. In this section, we’ll delve into the darker side of AI-driven recommendations and how businesses are addressing these issues.
A. Data Privacy and Security
Recommendation engines thrive on data, and lots of it. However, the collection and use of personal data raise concerns about privacy and security. Customers are increasingly aware of the data they share online and expect it to be handled responsibly.
To address these concerns, e-commerce platforms must implement robust data privacy policies and security measures. This includes transparent data collection practices, secure storage, and strict access controls. Complying with data protection regulations like GDPR and CCPA is not just a legal requirement; it’s a trust-building exercise with customers.
B. Bias in AI Recommendations
AI systems, including recommendation engines, are only as good as the data they are trained on. This opens the door to the possibility of bias. If historical data contains biases, AI can inadvertently perpetuate them, leading to unfair or discriminatory recommendations.
Businesses must actively work to identify and rectify bias in their recommendation algorithms. This involves auditing the data used for training, diversifying datasets, and regularly evaluating the fairness of recommendations. Ethical AI practices ensure that recommendation engines serve all users equally and without discrimination.
C. Maintaining Human Touch in E-commerce
While AI-driven recommendations are incredibly efficient, there’s a risk of losing the human touch in the e-commerce experience. Some users still prefer interacting with a knowledgeable salesperson or value the serendipity of discovering new products on their own.
Balancing AI automation with human interaction is essential. Offering options for users to seek help or engage with customer support humanizes the digital shopping experience. AI should enhance, not replace, the customer-service aspect of e-commerce.
Implementing AI Marketing and Recommendation Engines
Understanding the theory behind AI marketing and recommendation engines is one thing; implementing them effectively is another. In this section, we’ll outline the steps and best practices for bringing AI into your e-commerce strategy.
A. Steps to Implement AI Marketing
- Data Collection and Analysis : Data is the lifeblood of AI marketing. Start by collecting user data ethically and responsibly. Analyze this data to gain insights into customer behavior, preferences, and pain points. Tools like Google Analytics, Kissmetrics, or HubSpot can help.
- Choosing the Right AI Tools : The AI marketing landscape is vast, with tools for various purposes. Select tools that align with your goals. For instance, if you’re looking to enhance email marketing, consider platforms like Mailchimp or SendGrid.
- Integration with E-commerce Platforms : Seamless integration is key to AI marketing success. Ensure that the AI tools you choose can easily integrate with your e-commerce platform, whether it’s Shopify, WooCommerce, or a custom solution.
B. Best Practices for Recommendation Engine Implementation
- Continuous Learning Algorithms: Recommendation engines should evolve with your customers. Implement algorithms that continuously learn from user behavior and adapt recommendations accordingly. This keeps your suggestions fresh and relevant.
- User Feedback Loop: Encourage users to provide feedback on recommendations. Did they find the suggestions helpful? Did they make a purchase based on a recommendation? Use this feedback to fine-tune your algorithms and improve the quality of suggestions.
- A/B Testing: Experimentation is crucial in optimizing recommendation engines. Conduct A/B tests to compare different algorithms or recommendation strategies. This data-driven approach helps you identify what works best for your audience.
C. Measuring Success: Key Metrics
To gauge the effectiveness of your AI marketing and recommendation efforts, track key metrics such as:
-
Conversion Rate: Measure how many visitors turn into customers after interacting with AI-driven recommendations.
-
Click-Through Rate (CTR): Analyze how often users click on recommended products or content.
-
Revenue Generated: Calculate the revenue directly attributed to AI-driven recommendations.
-
Customer Retention: Monitor whether personalized recommendations lead to repeat purchases and increased customer loyalty.
-
Bounce Rate: Assess whether recommendations reduce the bounce rate, indicating that users are finding relevant content.
-
Customer Satisfaction: Collect feedback from users to gauge their satisfaction with the personalized shopping experience.
Using these metrics, you can fine-tune your AI marketing and recommendation strategies for optimal results.
Future Trends and Innovations
The world of e-commerce is ever-evolving, and AI continues to be at the forefront of innovation. In this section, we’ll explore some of the future trends and cutting-edge developments that will shape the e-commerce landscape.
A. AI-Powered Virtual Shopping Assistants
Imagine having a virtual shopping assistant that not only understands your preferences but can also engage in natural conversations, answer questions, and provide recommendations in real-time. AI-powered virtual shopping assistants are the future of personalized shopping experiences. These virtual companions will use Natural Language Processing (NLP) to understand and interact with customers, making online shopping feel as personal as a visit to a physical store.
B. Augmented Reality in E-commerce
Augmented Reality (AR) is poised to revolutionize how consumers shop online. AR allows users to visualize products in their real-world environment before making a purchase. For example, you can see how a piece of furniture fits in your living room or how a pair of shoes looks on your feet. This technology bridges the gap between online and offline shopping experiences, reducing uncertainty and increasing confidence in purchasing decisions.
C. Voice Search Optimization
With the rise of voice-activated virtual assistants like Siri and Alexa, voice search is becoming increasingly popular. E-commerce businesses will need to optimize their websites and product listings for voice search queries. This includes understanding conversational search patterns and providing concise, voice-friendly product information.
The future of e-commerce is undoubtedly exciting, with AI poised to play a pivotal role in shaping these innovations.
In conclusion, the world of e-commerce has evolved tremendously, thanks to the transformative power of AI marketing and recommendation engines. We’ve witnessed how AI-driven personalization, predictive analytics, and dynamic pricing have enhanced the shopping experience. Recommendation engines, whether collaborative filtering or content-based, have become indispensable in guiding customers to products they’ll love.
However, this transformation is not without its challenges, including data privacy, bias, and the need to strike the right balance between automation and human interaction. Implementing AI marketing and recommendation engines requires careful planning, the right tools, and a commitment to ethical practices.
As we look to the future, we anticipate even more exciting developments in the form of AI-powered virtual shopping assistants, augmented reality integration, and the optimization of e-commerce for voice search. These innovations will continue to push the boundaries of what’s possible in online shopping.
In this dynamic landscape, one thing is clear: businesses that embrace AI and adapt to changing consumer expectations will not only survive but thrive in the ever-evolving world of e-commerce.