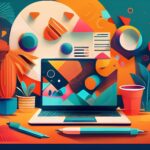
Cost-Effective AI Marketing Solutions for Startups
July 16, 2024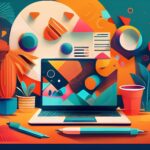
The Role of SEO in B2B Lead Generation: Optimizing Your Website for Success
July 18, 2024Predictive analytics has emerged as a powerful tool in the modern business landscape, enabling companies to anticipate future trends, optimize operations, and make data-driven decisions. By harnessing the power of big data and machine learning algorithms, businesses across various industries are transforming their strategies and achieving remarkable success. In this comprehensive guide, we explore real-world applications of predictive analytics and share inspiring success stories that highlight its transformative impact.
Introduction
In an era where data is often referred to as the new oil, the ability to predict future outcomes based on historical data has become a game-changer for businesses. Predictive analytics, a branch of advanced analytics that uses statistical techniques, machine learning, and artificial intelligence to analyze current and historical data, helps businesses forecast future events, behaviors, and trends. This capability allows companies to make proactive decisions, reduce risks, and seize new opportunities. In this article, we delve into how predictive analytics is revolutionizing industries and share success stories that demonstrate its real-world applications.
Understanding Predictive Analytics
Predictive analytics involves using data, statistical algorithms, and machine learning techniques to identify the likelihood of future outcomes based on historical data. The goal is to go beyond knowing what has happened to providing a best assessment of what will happen in the future. Here’s how it works:
Data Collection
The first step in predictive analytics is gathering data from various sources. This data can include customer information, transaction records, social media interactions, sensor data, and more.
Data Preparation
Once collected, the data must be cleaned and prepared for analysis. This involves removing any inconsistencies, filling in missing values, and transforming the data into a suitable format for analysis.
Model Building
Using statistical techniques and machine learning algorithms, predictive models are built to analyze the data and identify patterns and trends. These models can be trained on historical data to predict future outcomes.
Validation and Testing
The predictive models are validated and tested to ensure their accuracy and reliability. This involves comparing the model’s predictions with actual outcomes and making adjustments as needed.
Deployment
Once validated, the predictive models can be deployed in real-world applications, where they continuously analyze new data and provide insights for decision-making.
Real-World Applications of Predictive Analytics
Predictive analytics is being applied across a wide range of industries, driving innovation and improving outcomes. Here are some notable applications:
Healthcare
In healthcare, predictive analytics is used to improve patient outcomes, optimize resource allocation, and reduce costs. For example, predictive models can identify patients at high risk of developing chronic conditions, enabling early intervention and personalized treatment plans. Additionally, hospitals use predictive analytics to manage patient flow, ensuring that resources such as beds and staff are allocated efficiently.
Retail
Retailers leverage predictive analytics to enhance customer experiences, optimize inventory management, and increase sales. By analyzing customer behavior and purchase history, retailers can predict future buying patterns and tailor marketing campaigns accordingly. Predictive analytics also helps retailers manage inventory levels, reducing stockouts and overstock situations.
Finance
In the finance industry, predictive analytics is used to detect fraud, manage risks, and improve customer service. Financial institutions analyze transaction data to identify suspicious activities and prevent fraud. Predictive models also help in assessing credit risk, allowing lenders to make informed decisions about loan approvals. Additionally, banks use predictive analytics to personalize customer interactions, offering products and services that match individual needs.
Manufacturing
Manufacturers use predictive analytics to optimize production processes, reduce downtime, and improve product quality. By analyzing data from sensors and machines, predictive models can forecast equipment failures and schedule maintenance before breakdowns occur. This proactive approach minimizes downtime and extends the lifespan of machinery. Predictive analytics also helps manufacturers optimize production schedules and reduce waste.
Marketing
Marketing professionals use predictive analytics to design more effective campaigns, allocate budgets efficiently, and enhance customer targeting. By analyzing data on customer behavior, preferences, and interactions, predictive models can identify the most promising leads and tailor marketing messages to resonate with specific audiences. This leads to higher conversion rates and better ROI on marketing spend.
Transportation and Logistics
In transportation and logistics, predictive analytics is used to optimize routes, reduce fuel consumption, and improve delivery times. Logistics companies analyze data on traffic patterns, weather conditions, and shipment histories to predict the most efficient routes and delivery schedules. This helps in reducing operational costs and enhancing customer satisfaction by ensuring timely deliveries.
Success Stories in Predictive Analytics
Healthcare: Predicting Patient Deterioration
A major hospital system implemented predictive analytics to monitor patients in real-time and predict potential deterioration. By analyzing data from electronic health records, vital signs, and lab results, the predictive model identified patients at risk of severe complications. This enabled healthcare providers to intervene early, improving patient outcomes and reducing hospital readmission rates.
Retail: Enhancing Customer Loyalty
A leading retail chain used predictive analytics to enhance its loyalty program. By analyzing customer purchase data and preferences, the retailer identified high-value customers and tailored personalized offers to them. This resulted in increased customer retention, higher sales, and improved customer satisfaction.
Finance: Fraud Prevention
A global financial institution implemented predictive analytics to combat fraud. By analyzing transaction data in real-time, the predictive model identified unusual patterns indicative of fraudulent activity. This allowed the institution to take immediate action, preventing significant financial losses and safeguarding customer accounts.
Manufacturing: Reducing Downtime
A large manufacturing company used predictive analytics to predict equipment failures and schedule maintenance proactively. By analyzing data from machine sensors, the predictive model forecasted when equipment was likely to fail and recommended maintenance schedules. This approach reduced unplanned downtime, improved production efficiency, and extended the lifespan of the machinery.
Marketing: Improving Campaign Effectiveness
A multinational corporation used predictive analytics to optimize its marketing campaigns. By analyzing customer data and predicting future behavior, the company identified the most effective channels and messages for reaching its target audience. This resulted in higher engagement rates, increased conversions, and a significant boost in revenue.
The Future of Predictive Analytics
The potential of predictive analytics is vast, and its applications are continually expanding. Here are some trends to watch:
Integration with AI and Machine Learning
The integration of predictive analytics with AI and machine learning will enhance its capabilities, enabling more accurate predictions and deeper insights. AI-powered predictive models can learn and adapt over time, improving their accuracy and relevance.
Real-Time Analytics
Advancements in technology are enabling real-time predictive analytics, where data is analyzed and predictions are made instantly. This is particularly valuable in industries such as healthcare and finance, where timely insights can significantly impact outcomes.
Enhanced Personalization
Predictive analytics will continue to drive personalization in various industries, from marketing to healthcare. By understanding individual preferences and behaviors, businesses can deliver highly personalized experiences that meet customers’ unique needs.
Ethical Considerations
As predictive analytics becomes more prevalent, addressing ethical considerations such as data privacy and bias will be crucial. Businesses must ensure that their predictive models are transparent, fair, and respectful of user privacy.
Expanded Use Cases
New use cases for predictive analytics are emerging across industries. From agriculture to energy, businesses are discovering innovative ways to leverage predictive insights to drive efficiency and growth.
How do businesses ensure the accuracy of predictive models?
Businesses ensure the accuracy of predictive models through rigorous validation and testing processes. This involves comparing the model’s predictions with actual outcomes and making necessary adjustments. Continuous monitoring and updating of models based on new data also help maintain accuracy over time.
What ethical considerations should be addressed in predictive analytics?
Ethical considerations in predictive analytics include data privacy, fairness, and transparency. Businesses must ensure that they collect and use data responsibly, avoid biases in their models, and provide clear explanations of how predictions are made to maintain user trust and comply with regulations.
How can small businesses benefit from predictive analytics?
Small businesses can benefit from predictive analytics by using it to better understand their customers, optimize inventory, and improve marketing strategies. By leveraging affordable predictive analytics tools and services, small businesses can gain valuable insights and compete more effectively in their markets.
What role does big data play in predictive analytics?
Big data is essential for predictive analytics as it provides the large volumes of diverse data needed to identify patterns and trends. The more data available, the more accurate and reliable the predictive models can be, enabling businesses to make well-informed decisions.
How can predictive analytics improve customer service?
Predictive analytics can improve customer service by anticipating customer needs and preferences, allowing businesses to provide personalized experiences. For example, predictive models can forecast customer issues and proactively offer solutions, enhancing satisfaction and loyalty.
Conclusion
Predictive analytics stands as a transformative force across various industries, offering the power to foresee future trends and make data-driven decisions. By leveraging historical data and advanced algorithms, businesses can optimize their operations, reduce risks, and unlock new opportunities. The real-world applications and success stories shared in this guide highlight the significant impact predictive analytics can have on healthcare, retail, finance, manufacturing, marketing, and transportation.
As we move forward, the integration of predictive analytics with artificial intelligence and machine learning will further enhance its capabilities, enabling more accurate and timely insights. The potential for real-time analytics, enhanced personalization, and expanded use cases will drive innovation and efficiency in ways previously unimaginable.
However, with great power comes great responsibility. Businesses must address ethical considerations, such as data privacy and bias, to ensure that predictive analytics is used fairly and transparently. By doing so, they can build trust with customers and stakeholders while reaping the benefits of this powerful tool.
In conclusion, predictive analytics is not just a technological advancement; it is a strategic asset that empowers businesses to navigate the complexities of the modern world with confidence. By embracing predictive analytics, companies can stay ahead of the curve, anticipate future trends, and achieve remarkable success. The journey of harnessing predictive analytics is filled with potential, and as more businesses adopt and refine their capabilities, the stories of success and innovation will continue to inspire and shape the future of industry.
FAQs
What is predictive analytics?
Predictive analytics is a branch of advanced analytics that uses statistical techniques, machine learning, and artificial intelligence to analyze current and historical data to forecast future events, behaviors, and trends.
How is predictive analytics used in healthcare?
In healthcare, predictive analytics is used to identify patients at high risk of developing chronic conditions, optimize resource allocation, and improve patient outcomes through early intervention and personalized treatment plans.
Can predictive analytics help in fraud prevention?
Yes, predictive analytics can help in fraud prevention by analyzing transaction data to identify unusual patterns indicative of fraudulent activity, allowing financial institutions to take immediate action and prevent losses.
What are the benefits of predictive analytics in marketing?
Predictive analytics in marketing helps businesses design more effective campaigns, allocate budgets efficiently, and enhance customer targeting, leading to higher conversion rates and better ROI on marketing spend.
What is the future of predictive analytics?
The future of predictive analytics includes integration with AI and machine learning, real-time analytics, enhanced personalization, ethical considerations, and expanded use cases across various industries.