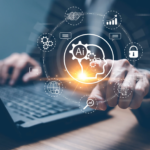
Chatbots, Predictive Analytics, and More: The AI Marketing Tools to Embrace in 2025
December 27, 2024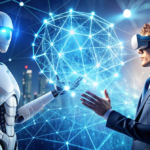
Understanding Machine Learning and Its Role in Marketing
December 30, 2024Introduction
Machine learning (ML) is no longer a futuristic concept confined to academic research or high-tech industries; it has become a transformative force across various sectors, including marketing. By enabling systems to learn and improve from data without explicit programming, ML empowers marketers to deliver highly targeted, efficient, and innovative campaigns. As businesses grapple with the explosion of data and the need for personalization, machine learning provides the tools to not only make sense of this data but to use it to create meaningful customer experiences.
This article explores the fundamentals of machine learning, its integration into marketing strategies, and how businesses can harness its potential. From predictive analytics to dynamic customer segmentation, the applications of ML in marketing are vast and continually evolving. Understanding these capabilities can help businesses stay ahead in an increasingly competitive landscape.
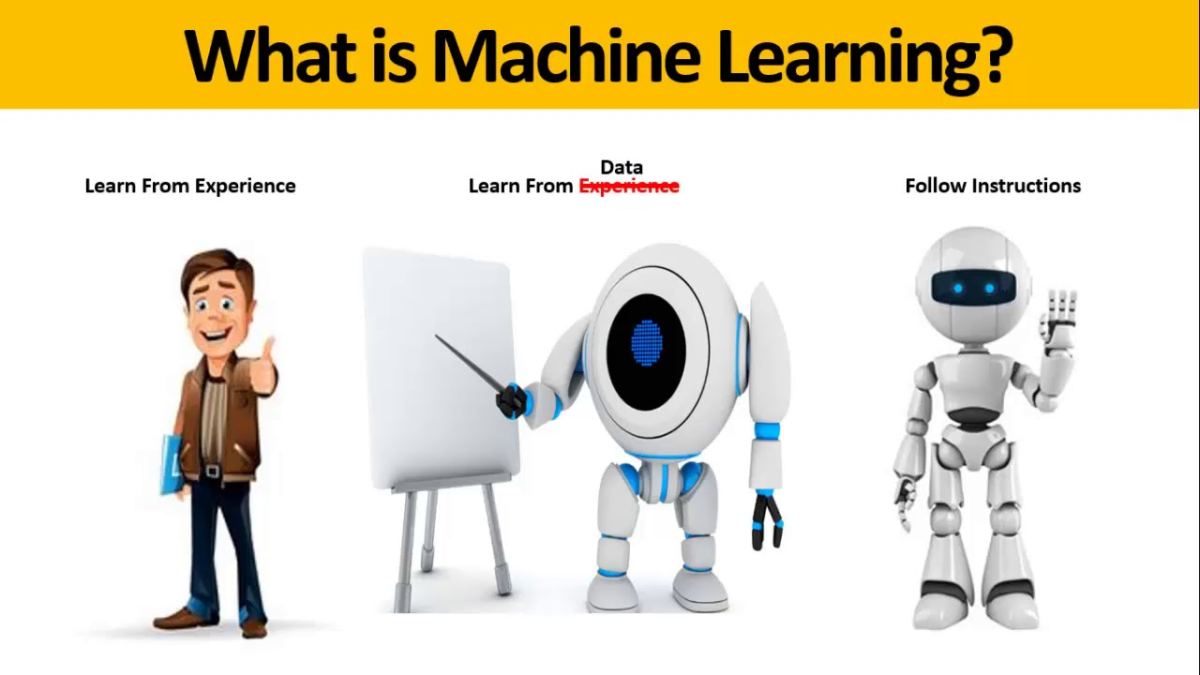
What is Machine Learning?
Machine learning is a subset of artificial intelligence (AI) focused on creating systems that can learn from and adapt to data. Unlike traditional programming, where explicit instructions are coded, ML algorithms analyze patterns in data to make decisions or predictions. These systems improve over time as they process more data, making them ideal for tasks that require adaptability and scale.
At its core, ML relies on three primary components:
- Data: The foundation of any ML model. This includes historical customer data, transaction records, website analytics, and more.
- Algorithms: These are mathematical models that analyze and process the data. Examples include linear regression, decision trees, and neural networks.
- Training: ML models require training using labeled datasets (supervised learning), unlabeled data (unsupervised learning), or a combination (semi-supervised learning).
It’s important to differentiate machine learning from related fields like AI and deep learning. While AI is the broader concept of machines simulating human intelligence, ML focuses on data-driven learning. Deep learning, a subset of ML, uses complex neural networks to mimic human brain function and is especially powerful for tasks like image recognition and natural language processing.
Examples of ML in everyday life are plentiful, from Netflix’s content recommendations and Google’s search algorithms to the personalized ads that populate social media feeds. These applications underscore the versatility of ML in processing vast amounts of data to deliver actionable insights.
Machine learning isn’t just a technical innovation—it’s a practical solution to many challenges marketers face today. Understanding its foundational elements can help businesses unlock its potential to improve customer engagement, streamline operations, and drive growth.
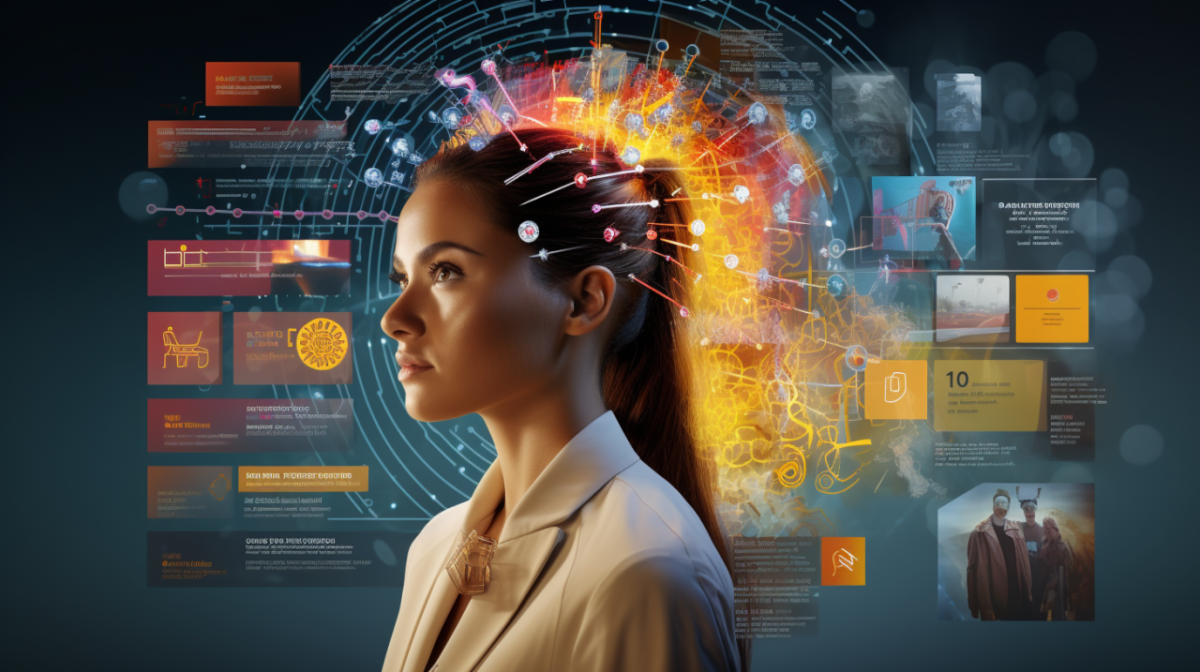
The Intersection of Machine Learning and Marketing
Marketing has always been about understanding customer needs and delivering value. Traditionally, this relied on manual data analysis, intuition, and broad segmentation. However, the digital revolution has exponentially increased the volume of data generated by customers—ranging from browsing behaviors and purchase histories to social media interactions and feedback. This data explosion has made manual methods inefficient, paving the way for machine learning (ML) to become an essential tool in modern marketing.
Machine learning transforms marketing by making it more data-driven, precise, and personalized. Unlike traditional methods that often rely on static assumptions, ML uses algorithms to analyze real-time data, detect patterns, and adapt strategies dynamically. For example, an e-commerce platform can leverage ML to recommend products tailored to individual preferences, improving both the customer experience and sales outcomes.
Another critical aspect is automation. Machine learning can handle tasks that were once time-consuming, such as customer segmentation or content personalization, at a scale that would be impossible for human marketers alone. This not only saves time but also ensures that marketing strategies remain relevant and effective as customer behaviors evolve.
Furthermore, ML enhances decision-making by providing predictive insights. Marketers can use ML-powered tools to forecast trends, anticipate customer needs, and identify high-value opportunities. For instance, predictive analytics might reveal which customers are most likely to churn, enabling targeted retention campaigns. This proactive approach significantly improves the efficiency and ROI of marketing efforts.
In essence, the intersection of machine learning and marketing marks a shift from guesswork to precision. By leveraging ML, marketers can harness the full potential of data, deliver hyper-personalized experiences, and achieve greater efficiency, making it a cornerstone of modern marketing strategies.
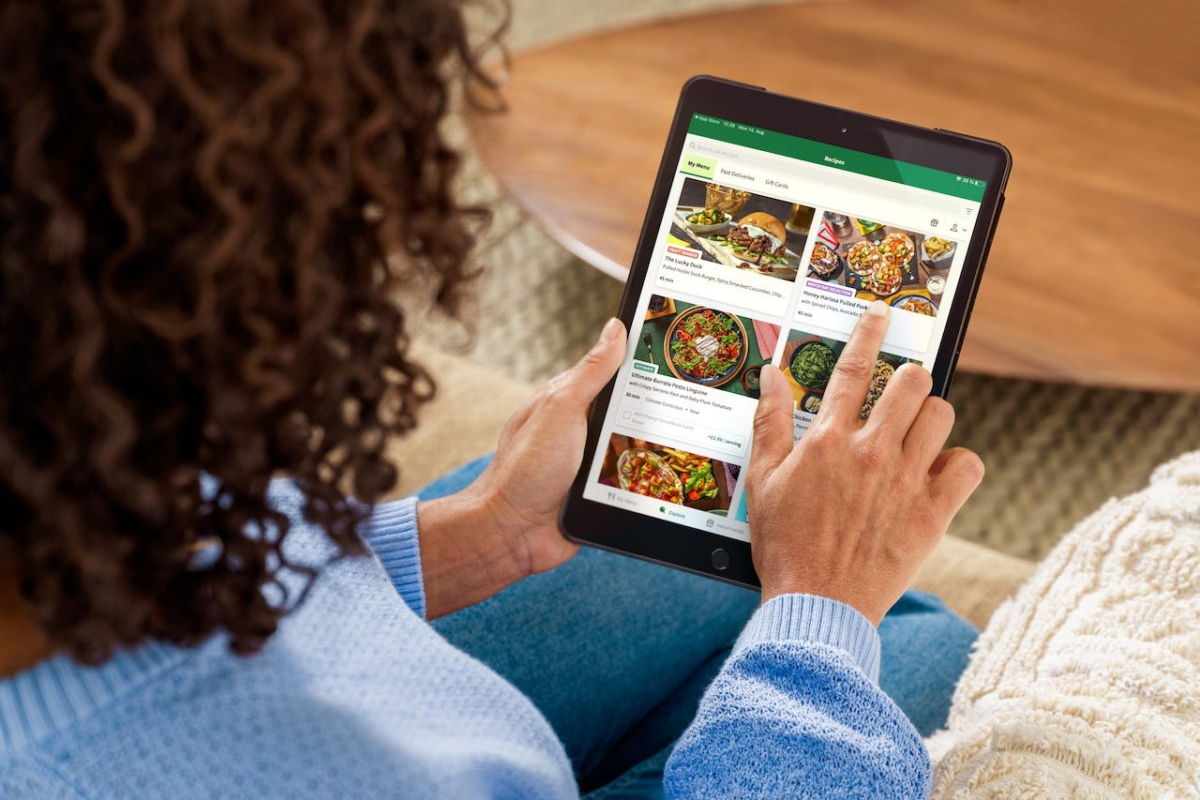
Key Applications of Machine Learning in Marketing
Machine learning has unlocked numerous applications in marketing, enabling businesses to achieve their goals with unprecedented accuracy and efficiency. Below are some of the most impactful ways ML is being used in marketing:
- Personalized Customer Experiences
One of the most visible applications of ML is in creating personalized customer experiences. Whether it’s product recommendations on Amazon or tailored playlists on Spotify, ML algorithms analyze individual preferences and behaviors to deliver highly relevant suggestions. This level of personalization fosters customer loyalty and increases engagement.
In email marketing, for instance, ML can predict the type of content a customer is most likely to interact with, ensuring that emails are not just opened but acted upon. By analyzing factors like past interactions, purchase history, and even the time of day a customer is most active, ML helps businesses optimize their outreach strategies.
- Predictive Analytics
Predictive analytics uses historical data to forecast future outcomes, and it has become a cornerstone of ML-driven marketing. By analyzing customer behavior patterns, predictive models can identify trends, anticipate needs, and guide decision-making.
For example, a retailer can use predictive analytics to determine which products are likely to sell during a particular season, allowing them to optimize inventory and marketing campaigns. Similarly, subscription-based businesses can use these insights to identify customers at risk of churning and implement retention strategies before it’s too late.
- Automated Customer Segmentation
Traditional customer segmentation often involves broad categories like age, gender, or location. Machine learning takes this a step further by analyzing behavioral and transactional data to create micro-segments. These segments are more accurate and actionable, enabling businesses to craft highly targeted campaigns.
For instance, a streaming service might identify a segment of users who prefer action movies but also show interest in documentaries. By targeting this group with mixed-content recommendations, they can maximize engagement. Automated segmentation ensures that marketing efforts are focused on the right audience, enhancing efficiency and results.
By integrating these applications, businesses can not only meet but exceed customer expectations, fostering stronger relationships and driving growth. Machine learning isn’t just enhancing marketing—it’s redefining it.
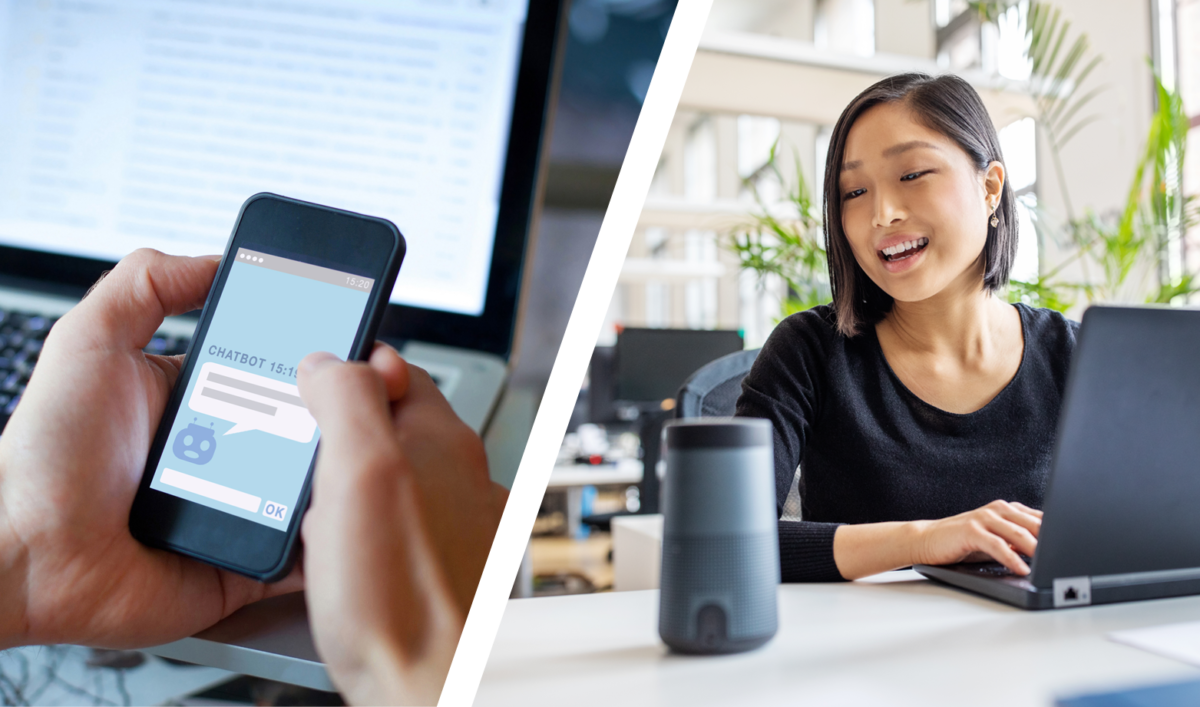
Chatbots and Virtual Assistants
One of the most transformative applications of machine learning (ML) in marketing is the use of chatbots and virtual assistants. These tools leverage natural language processing (NLP), a subset of ML, to interact with customers in a human-like manner, providing instant responses, personalized recommendations, and efficient problem resolution.
Chatbots are now a common feature on websites and social media platforms, assisting customers with inquiries ranging from product information to order tracking. For example, a retail website can use a chatbot to guide customers through the shopping process, offering tailored product suggestions based on browsing behavior and purchase history. These interactions are not only convenient for customers but also help businesses capture valuable data to refine their marketing strategies.
Virtual assistants like Siri, Alexa, and Google Assistant go a step further by integrating with other applications and devices. They can perform tasks such as booking appointments, sending reminders, or even making purchases on behalf of users. Marketers can optimize their content to be compatible with these assistants, ensuring their products and services are discoverable through voice search—a rapidly growing trend.
Beyond customer service, chatbots and virtual assistants are powerful tools for lead generation and nurturing. By engaging with potential customers in real time, they can collect contact information, answer preliminary questions, and schedule follow-ups. This automation streamlines the sales funnel, allowing marketing teams to focus on high-value tasks.
The integration of ML into chatbots and virtual assistants has elevated customer interaction from basic query resolution to sophisticated, personalized engagement. These tools not only enhance user experience but also drive efficiency and scalability in marketing efforts.
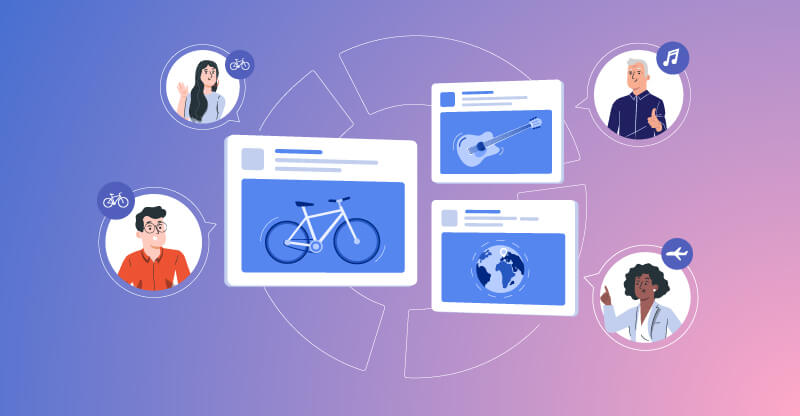
Ad Targeting and Optimization
Advertising has always been a critical component of marketing, but machine learning has revolutionized how ads are targeted and optimized. With ML, businesses can analyze vast amounts of data to identify the right audience, the best timing, and the most effective messaging for their ads.
One of the most significant advancements is in programmatic advertising, where ML algorithms automate the buying and placement of ads in real time. This process involves real-time bidding (RTB), where ad space is auctioned to the highest bidder within milliseconds. ML ensures that ads are displayed to the most relevant audience, maximizing the return on ad spend (ROAS).
For instance, if a customer frequently searches for fitness equipment, ML algorithms can identify this interest and serve them ads for related products, such as yoga mats or dumbbells. By analyzing factors like search history, demographics, and browsing patterns, ML creates highly targeted ad campaigns that are more likely to convert.
ML also plays a crucial role in ad optimization. It continuously monitors campaign performance, adjusting parameters such as budget allocation, keywords, and placement to improve outcomes. For example, if an ad is underperforming on one platform but excelling on another, ML algorithms can automatically reallocate resources to maximize effectiveness.
Moreover, predictive analytics in ML can forecast which ads are likely to perform well based on historical data. This allows marketers to test and refine their strategies before launching full-scale campaigns, saving time and resources.
In the realm of digital marketing, ML-driven ad targeting and optimization ensure that every dollar spent contributes to measurable results. By delivering the right message to the right audience at the right time, businesses can achieve higher engagement, conversion rates, and customer satisfaction.
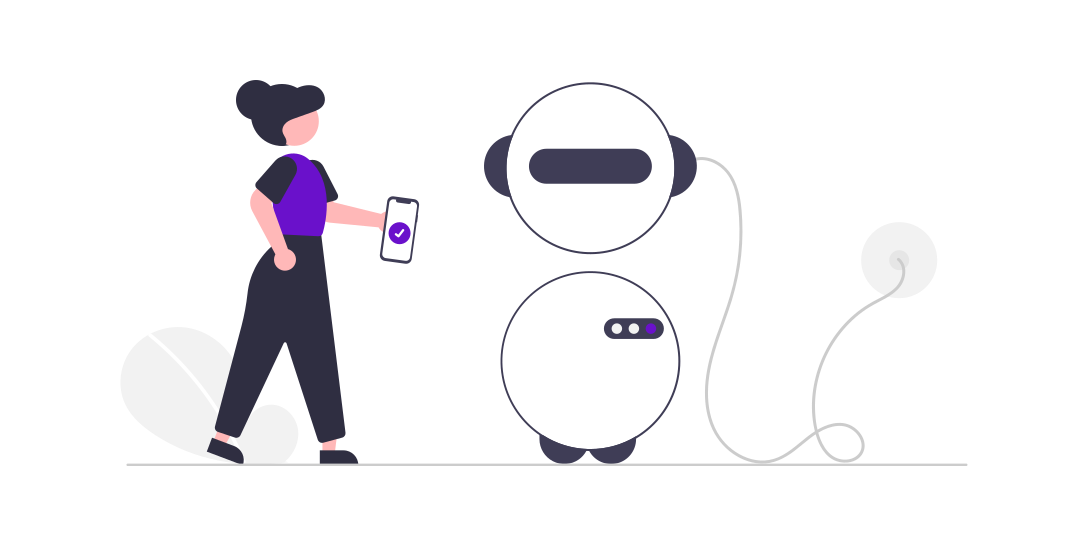
Benefits of Machine Learning in Marketing
The integration of machine learning (ML) into marketing offers numerous benefits that transform the way businesses interact with their customers and achieve their goals. These advantages not only enhance efficiency but also enable marketers to deliver value in innovative and impactful ways.
- Enhanced Decision-Making
One of the primary benefits of ML is its ability to process and analyze vast amounts of data quickly and accurately. By identifying patterns and trends, ML provides actionable insights that empower marketers to make informed decisions. For instance, an ML-powered dashboard might highlight which customer segments are most profitable, enabling businesses to allocate resources effectively. - Cost-Effectiveness and Efficiency
Automation is a hallmark of ML applications in marketing. Tasks that traditionally required manual effort, such as data analysis, segmentation, and content personalization, can now be completed in a fraction of the time. This not only reduces operational costs but also frees up marketing teams to focus on strategic initiatives. For example, automated email campaigns powered by ML can run continuously with minimal oversight, ensuring consistent customer engagement. - Improved Customer Satisfaction and Loyalty
ML enables hyper-personalized experiences, which are crucial for building strong customer relationships. By understanding individual preferences and behaviors, businesses can deliver tailored recommendations, targeted offers, and relevant content. Customers are more likely to remain loyal to brands that anticipate their needs and provide value. For instance, a subscription service using ML to recommend products based on previous purchases can significantly enhance customer satisfaction. - Scalability
As businesses grow, maintaining personalized interactions with a large customer base becomes challenging. ML provides scalability by automating complex processes without compromising on quality. Whether it’s managing a global advertising campaign or handling customer inquiries through chatbots, ML ensures that businesses can scale their operations seamlessly.
The benefits of ML in marketing are undeniable. By enhancing decision-making, improving efficiency, and fostering customer loyalty, ML positions businesses for long-term success in an increasingly competitive marketplace.
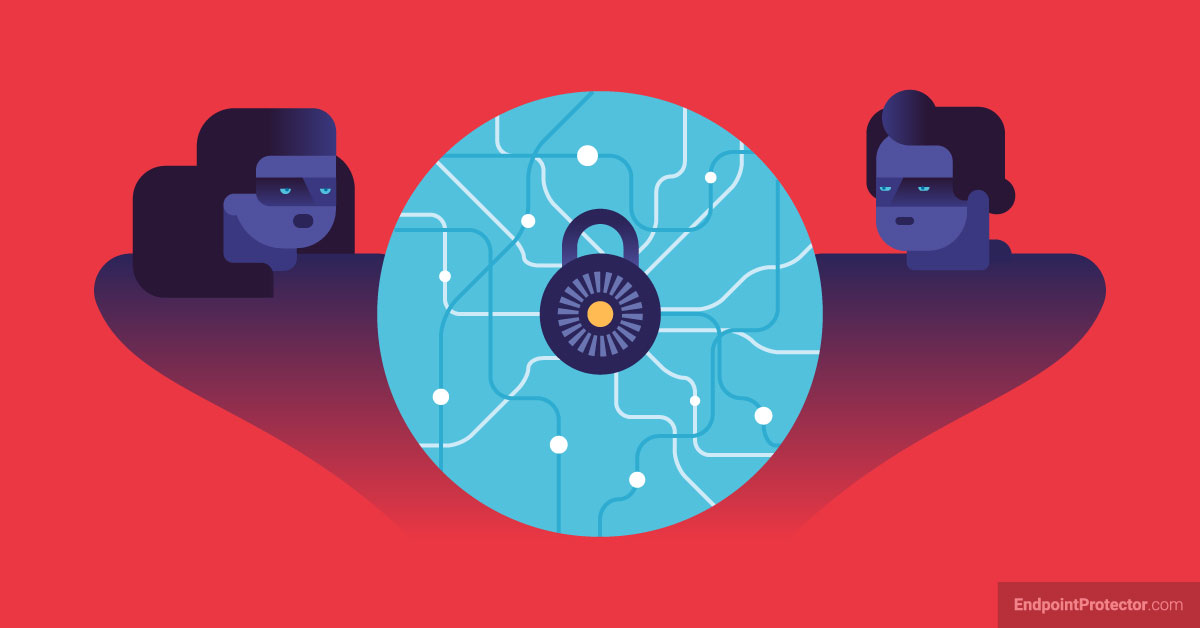
Challenges of Integrating Machine Learning in Marketing
While the advantages of machine learning (ML) in marketing are significant, its implementation is not without challenges. Businesses must navigate these obstacles carefully to fully harness ML’s potential.
- Data Privacy Concerns and Compliance
One of the most pressing challenges is ensuring compliance with data privacy regulations such as the General Data Protection Regulation (GDPR) and the California Consumer Privacy Act (CCPA). ML relies heavily on data, but mishandling this data can lead to legal repercussions and loss of customer trust. Marketers must strike a balance between leveraging data for personalization and respecting privacy. Implementing robust data security measures and obtaining explicit customer consent are critical steps. - Dependence on Quality Data
The accuracy and effectiveness of ML models depend on the quality of the data they process. Incomplete, outdated, or biased data can lead to flawed predictions and suboptimal outcomes. For example, if a dataset lacks diversity, ML algorithms may perpetuate biases, resulting in marketing strategies that alienate certain customer groups. Ensuring data integrity through regular audits and updates is essential. - Complexity and Resource Requirements
Integrating ML into marketing requires technical expertise, infrastructure, and investment. Smaller businesses may struggle to afford the tools and talent needed for successful implementation. Additionally, training ML models and maintaining them over time can be resource-intensive. Businesses must assess their readiness and consider partnering with third-party providers to mitigate these challenges. - Ethical Considerations in AI-Driven Marketing
The use of ML in marketing raises ethical questions about transparency and manipulation. For instance, predictive analytics can anticipate customer vulnerabilities, but exploiting these insights for profit can damage a brand’s reputation. Marketers must prioritize ethical practices and maintain transparency in their use of ML.
Despite these challenges, businesses that approach ML integration strategically can overcome these hurdles and unlock its transformative potential. By addressing issues like data privacy, quality, and ethics, companies can build a solid foundation for sustainable growth powered by machine learning.
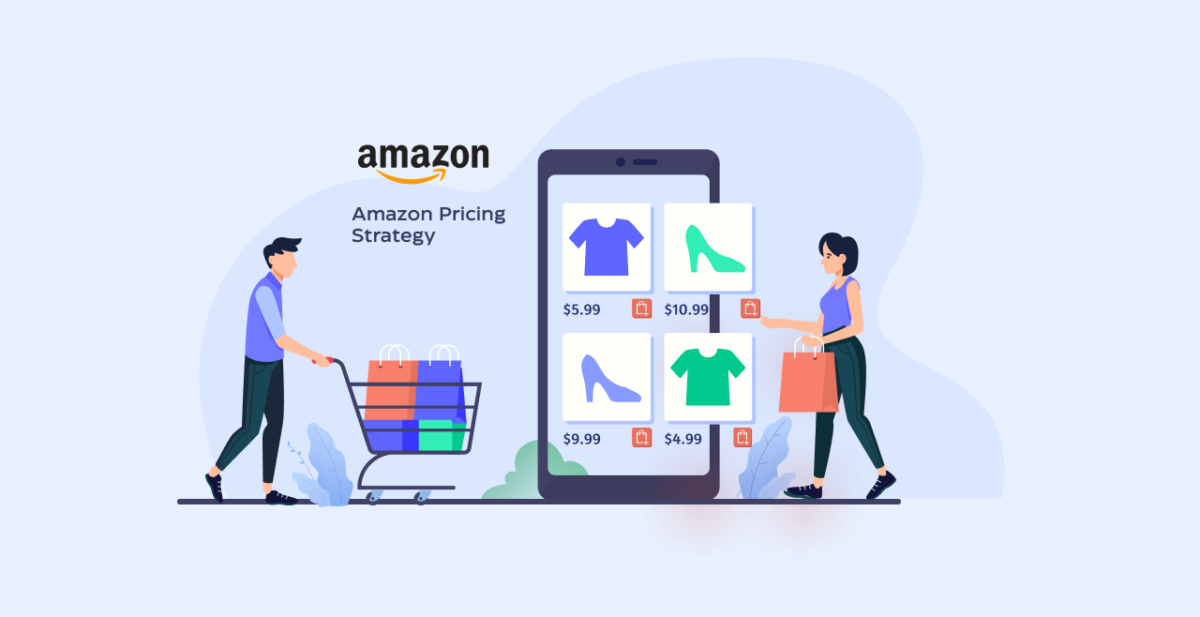
Case Studies
Real-world applications of machine learning (ML) in marketing provide a glimpse into its transformative potential. Below are three compelling case studies showcasing how leading brands have leveraged ML to achieve remarkable outcomes.
- Netflix’s Personalized Recommendations
Netflix is a prime example of how ML can revolutionize customer engagement. The streaming giant uses sophisticated ML algorithms to analyze viewing habits, search patterns, and user ratings. Based on this data, it generates personalized recommendations tailored to individual preferences.
For instance, if a user frequently watches documentaries and rates them highly, Netflix’s ML model prioritizes suggesting similar content. This personalization not only enhances user satisfaction but also increases the likelihood of continued subscriptions. Netflix estimates that its recommendation engine saves the company over $1 billion annually by reducing churn and improving user retention.
- Amazon’s Dynamic Pricing Strategies
Amazon’s success as a retail behemoth is partly due to its innovative use of ML in dynamic pricing. The company’s algorithms continuously analyze factors such as demand, competitor pricing, inventory levels, and customer behavior to adjust prices in real time.
For example, during peak shopping seasons like Black Friday, Amazon’s ML models identify trends and optimize prices to attract more customers while maximizing profit margins. This strategy ensures competitiveness and enhances customer trust in finding the best deals.
- Coca-Cola’s AI-Powered Campaigns
Coca-Cola has embraced ML to drive creativity and engagement in its marketing campaigns. By analyzing social media data, customer feedback, and sales trends, the company tailors its campaigns to resonate with target audiences.
A notable example is Coca-Cola’s use of AI to generate content for its “Share a Coke” campaign. By analyzing customer names and preferences, the company created personalized bottles and digital ads, resulting in a significant boost in sales and brand awareness. This campaign highlights how data-driven creativity powered by ML can deliver impactful results.
These case studies illustrate the versatility of ML in addressing diverse marketing challenges, from personalization and pricing to creative engagement. Businesses of all sizes can draw inspiration from these examples to innovate and thrive in their marketing efforts.
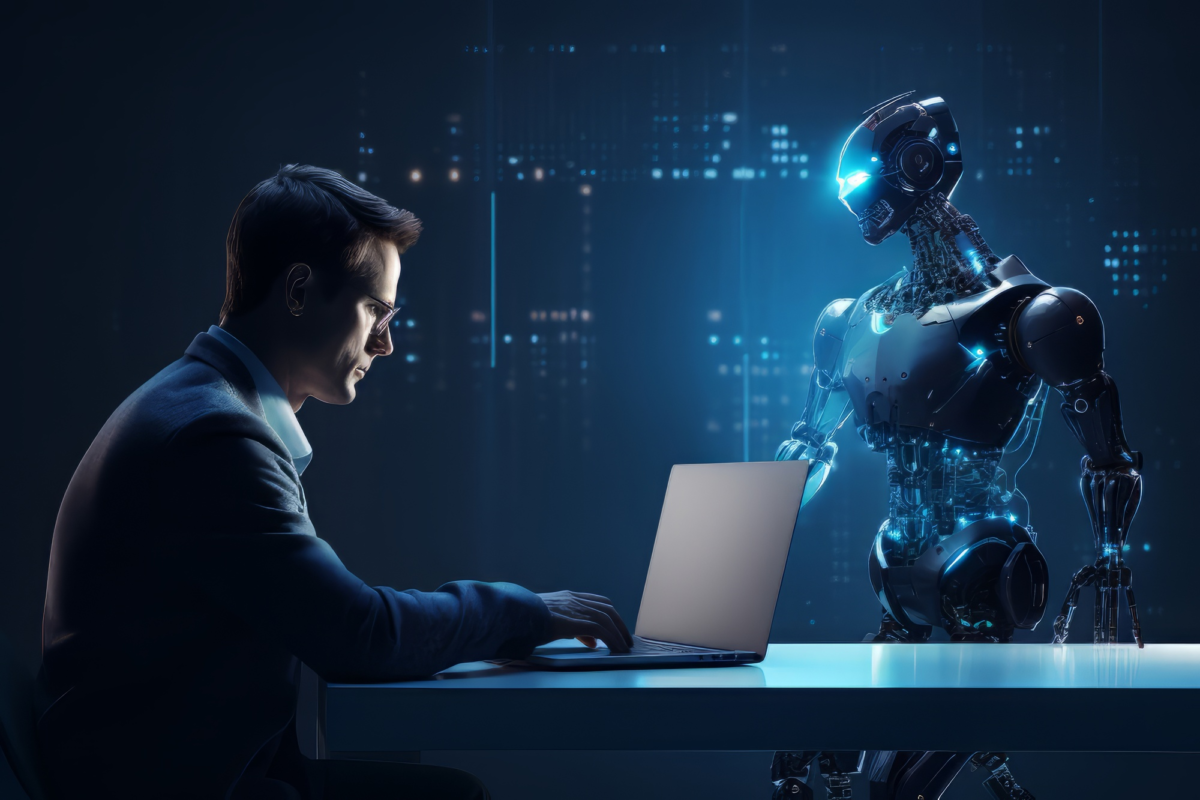
The Future of Machine Learning in Marketing
The role of machine learning (ML) in marketing is set to expand further as technology evolves. Emerging trends and innovations promise to redefine the marketing landscape, making it more intelligent, personalized, and efficient.
- Conversational AI and Hyper-Personalization
Conversational AI, powered by advanced ML models, is poised to take customer interactions to the next level. Tools like chatbots and virtual assistants are becoming more intuitive, capable of understanding context and emotions to deliver meaningful responses. This advancement paves the way for hyper-personalization, where every interaction is tailored to individual preferences and needs.
For instance, future e-commerce platforms might use ML to create virtual shopping assistants that guide customers through personalized product recommendations in real time, mimicking the experience of an in-store consultation.
- Omnichannel Marketing Integration
ML will play a crucial role in unifying omnichannel marketing strategies. By analyzing data across various touchpoints—websites, social media, email, and physical stores—ML can create a seamless customer journey. For example, a customer who browses a product online might receive a personalized discount code via email, followed by a targeted ad on social media, ensuring consistent engagement across channels. - Ethical and Transparent AI Practices
As ML becomes more pervasive, ethical considerations will gain prominence. Businesses will need to prioritize transparency in how they use ML to process data and make decisions. Regulations and guidelines may emerge to ensure that ML applications in marketing are fair, unbiased, and respectful of privacy. - AI-Generated Content and Creativity
ML is already being used to create content, from automated product descriptions to AI-generated artwork. In the future, this capability will become more sophisticated, allowing marketers to produce high-quality, customized content at scale. This innovation will free up creative teams to focus on strategy and storytelling.
The future of machine learning in marketing is bright, with endless possibilities for innovation and growth. By staying ahead of these trends, businesses can position themselves as leaders in the ever-evolving digital landscape.
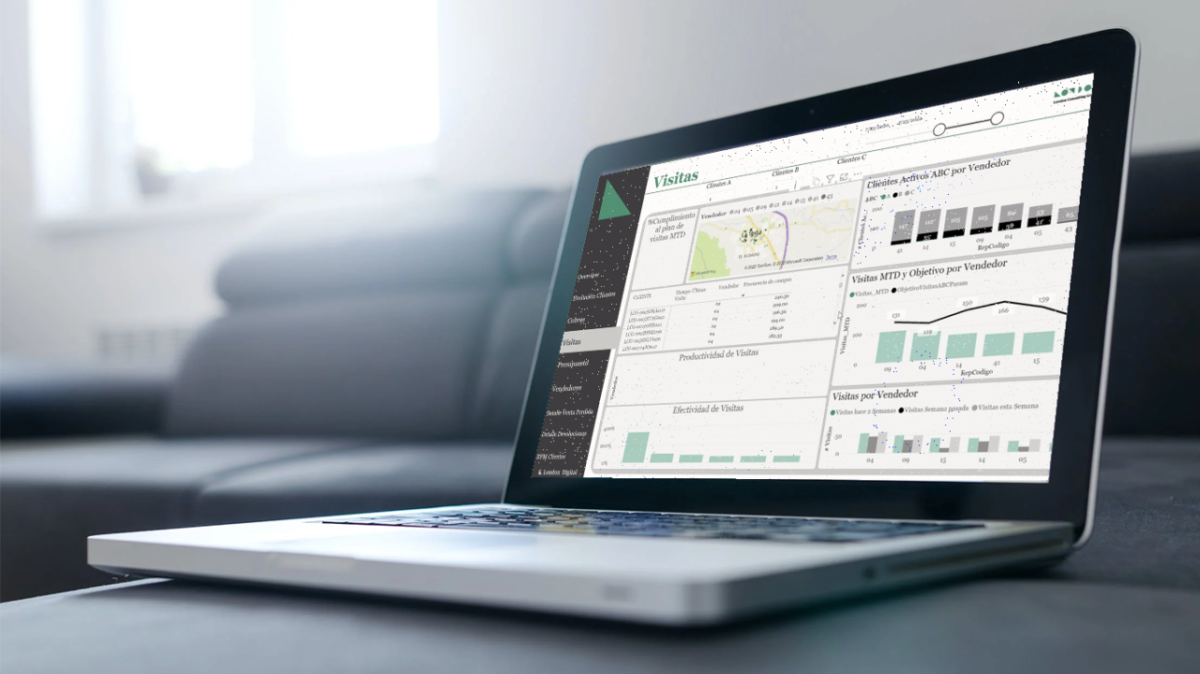
Practical Steps for Marketers to Get Started with Machine Learning
While machine learning (ML) offers transformative potential for marketing, getting started can feel overwhelming, especially for businesses new to the technology. However, with a strategic approach, marketers can successfully integrate ML into their operations and achieve meaningful results. Here are practical steps to begin leveraging ML in marketing:
- Identify Clear Goals and Opportunities
Before implementing ML, it’s essential to define specific objectives. Are you looking to improve customer segmentation, enhance ad targeting, or automate repetitive tasks? Clear goals provide direction and help in selecting the right tools and technologies.
For example, a retailer might aim to reduce cart abandonment rates. In this case, ML-powered predictive analytics can identify patterns leading to abandonment, enabling targeted interventions like personalized follow-up emails or discounts.
- Leverage Existing Data
Data is the backbone of ML. Start by auditing your current data sources, such as CRM systems, website analytics, and social media platforms. Ensure the data is clean, relevant, and comprehensive. If gaps exist, consider strategies to collect more meaningful customer data, such as surveys or incentivized feedback forms.
For instance, a travel agency might use historical booking data to train an ML model that predicts peak travel periods and recommends personalized offers.
- Choose the Right Tools and Platforms
Numerous ML tools and platforms cater to marketing needs, ranging from beginner-friendly solutions like Google Analytics and HubSpot to advanced platforms like IBM Watson and TensorFlow. Evaluate these options based on your goals, budget, and technical expertise.
If your team lacks technical skills, consider partnering with a third-party provider or leveraging no-code platforms that simplify ML implementation. These tools allow marketers to build predictive models and automate workflows without extensive coding knowledge.
- Build a Cross-Functional Team
Successful ML integration often requires collaboration between marketing, data science, and IT teams. If in-house expertise is limited, hiring or consulting with ML specialists can accelerate implementation and ensure accuracy. - Start Small and Scale Gradually
Begin with a pilot project to test the effectiveness of ML in a specific area. Monitor results, refine the model, and expand its application once you’re confident in its performance. For example, start with an ML-driven email campaign before scaling to include personalized website content and dynamic pricing.
By following these steps, marketers can overcome initial barriers and unlock the full potential of machine learning, ensuring a competitive edge in a data-driven world.
Conclusion
Machine learning (ML) has emerged as a game-changer in the marketing landscape, offering unprecedented opportunities for personalization, efficiency, and innovation. From predictive analytics and automated segmentation to chatbots and ad optimization, ML is reshaping how businesses engage with customers and achieve their goals.
While the journey to ML adoption comes with challenges—such as data privacy concerns and resource requirements—these obstacles are manageable with a strategic approach. By defining clear goals, leveraging quality data, and starting small, marketers can successfully integrate ML into their operations and drive significant results.
The future of marketing lies in data-driven decision-making, and machine learning is the key to unlocking its full potential. Businesses that embrace this technology today will not only stay ahead of the competition but also build deeper, more meaningful connections with their customers.